Sixfold Content
Product Update
Meet the First AI Accuracy Validator Built for Insurance Underwriting
Today, we’re excited to introduce the first-ever AI Accuracy Validator built for insurance underwriting. This application provides customers with a transparent and comprehensive way to evaluate Sixfold’s accuracy—reinforcing our commitment to bring reliable and trustworthy risk assessments to underwriters.

Stay informed, gain insights, and elevate your understanding of AI's role in the insurance industry with our comprehensive collection of articles, guides, and more.
.png)
AI Vendor Compliance: A Practical Guide for Insurers
In the hands of insurers, AI can drive great efficiency —safely and responsibly. We recently sat down with Matt Kelly, Data Strategy & Security expert and counsel at Debevoise & Plimpton, to explore how insurers can achieve this.
In the hands of insurers, AI can drive great efficiency —safely and responsibly. We recently sat down with Matt Kelly, Data Strategy & Security expert and counsel at Debevoise & Plimpton, to explore how insurers can achieve this.
Matt has played a key role in developing Sixfold’s 2024 Responsible AI Framework. With deep expertise in AI governance, he has led a growing number of insurers through AI implementations as adoption accelerates across the insurance industry.
To support insurers in navigating the early stages of compliance evaluation, he outlined four key steps:
Step 1: Define the Type of Vendor
Before getting started, it’s important to define what type of AI vendor you’re dealing with. Vendors come in various forms, and each type serves a different purpose. Start by asking these key questions:
- Are they really an AI vendor at all? Practically all vendors use AI (or will do so soon) – even if only in the form of routine office productivity tools and CRM suites. The fact that a vendor uses AI does not mean they use it in a way that merits treating them as an “AI vendor.” If the vendor’s use of AI is not material to either the risk or value proposition of the service or software product being offered (as may be the case, for instance, if a vendor uses it only for internal idea generation, background research, or for logistical purposes), ask yourself whether it makes sense to treat them as an AI vendor at all.
- Is this vendor delivering AI as a standalone product, or is it part of a broader software solution? You need to distinguish between vendors that are providing an AI system that you will interact with directly, versus those who are providing a software solution that leverages AI in a way that is removed from any end users.
- What type of AI technology does this vendor offer? Are they providing or using machine learning models, natural language processing tools, or something else entirely? Have they built or fine-tuned any of their AI systems themselves, or are they simply built atop third-party solutions?
- How does this AI support the insurance carrier’s operations? Is it enhancing underwriting processes, improving customer service, or optimizing operational efficiency?
Pro Tip: Knowing what type of AI solution you need and what the vendor provides will set the stage for deeper evaluations. Map out a flowchart of potential vendors and their associated risks.
Step 2: Identify the Risks Associated with the Vendor
Regulatory and compliance risks are always present when evaluating AI vendors, but it’s important to understand the specific exposures for each type of implementation. Some questions to consider are:
- Are there specific regulations that apply? Based on your expected use of the vendor, are there likely to be specific regulations that would need to be satisfied in connection with the engagement (as would be the case, for instance, with using AI to assist with underwriting decisions in various jurisdictions)?
- What are the data privacy risks? Does the vendor require access to sensitive information – particularly personal information or material nonpublic information – and if so, how do they protect it? Can a customer’s information easily be removed from the underlying AI or models?
- How explainable are their AI models? Are the decision-making processes clear, are they well documented, and can the outputs be explained to and understood by third parties if necessary?
- What cybersecurity protocols are in place? How does the vendor ensure that AI systems (and your data) are secure from misuse or unauthorized access?
- How will things change? What has the vendor committed to do in terms of ongoing monitoring and maintenance? How will you monitor compliance and consistency going forward?
Pro Tip: A good approach is to create a comprehensive checklist of potential risks for evaluation. For each risk that can be addressed through contract terms, build a playbook that includes key diligence questions, preferred contract clauses, and acceptable backup options. This will help ensure all critical areas are covered and allow you to handle each risk with consistency and clarity.
Step 3: Evaluate How Best to Mitigate the Identified Risks
Your company likely has processes in place to handle third-party risks, especially when it comes to data protection, vendor management, and quality control. However, not all risks may be covered, and they may need new or different mitigations. Start by asking:
- What existing processes already address AI vendor risks? For example, if you already have robust data privacy policies, consider whether those policies cover key AI-related risks, and if so, ensure they are incorporated into the AI vendor review process.
- Which risks remain unresolved? Identify the gaps in your current processes to identify unique residual risks – such as algorithmic biases or the need for external audits on AI models – that will require new and ongoing resource allocations.
- How can we mitigate the residual risks? Rather than relying solely on contractual provisions and commercial remedies, consider alternative methods to mitigate residual risks, including data access controls and other technical limitations. For instance, when it comes to sharing personal or other protected data, consider alternative means (including the use of anonymized, pseudonymized, or otherwise abstracted datasets) to help limit the exposure of sensitive information.
Pro Tip: You don’t always need to reinvent the wheel. Look at existing processes within your organization, such as those for data privacy, and determine if they can be adapted to cover AI-specific risks.
Step 4: Establish a Plan for Accepting and Governing Remaining Risks
Eliminating all AI vendor risks cannot be the goal. The goal must be to identify, measure, and mitigate AI vendor risks to a level that is reasonable and that can be accepted by a responsible, accountable person or committee. Keep these final considerations in mind:
- How centralized is your company’s decision-making process? Some carriers may have a centralized procurement team handling all AI vendor decisions, while others may allow business units more autonomy. Understanding this structure will guide how risks are managed.
- Who is accountable for evaluating and approving these risks? Should this decision be made by a procurement team, the business unit, or a senior executive? Larger engagements with greater risks may require involvement from higher levels of the company.
- Which risks are too significant to be accepted? In any vendor engagement, some risks may simply be unacceptable to the carrier. For example, allowing a vendor to resell policyholder information to third parties would often fall into this category. Those overseeing AI vendor risk management usually identify these types of risks instinctively, but clearly documenting them helps ensure alignment among all stakeholders, including regulators and affected parties.
One-Process-Fits-All Doesn’t Apply
As AI adoption grows in insurance, taking a strategic approach can help simplify review processes and prioritize efforts. These four steps provide the foundation for making informed, secure decisions from the start of your AI implementation project.
Evaluating AI vendors is a unique process for each carrier that requires clarity about the type of vendor, understanding the risks, identifying the gaps in your existing processes, and deciding how to mitigate the remaining risks moving forward. Each organization will have a unique approach based on its structure, corporate culture, and risk tolerance.
“Every insurance carrier that I’ve worked with has its own unique set of tools and rules for evaluating AI vendors, what works for one may not be the right fit for another.”
- Matt Kelly, Counsel at Debevoise & Plimpton.
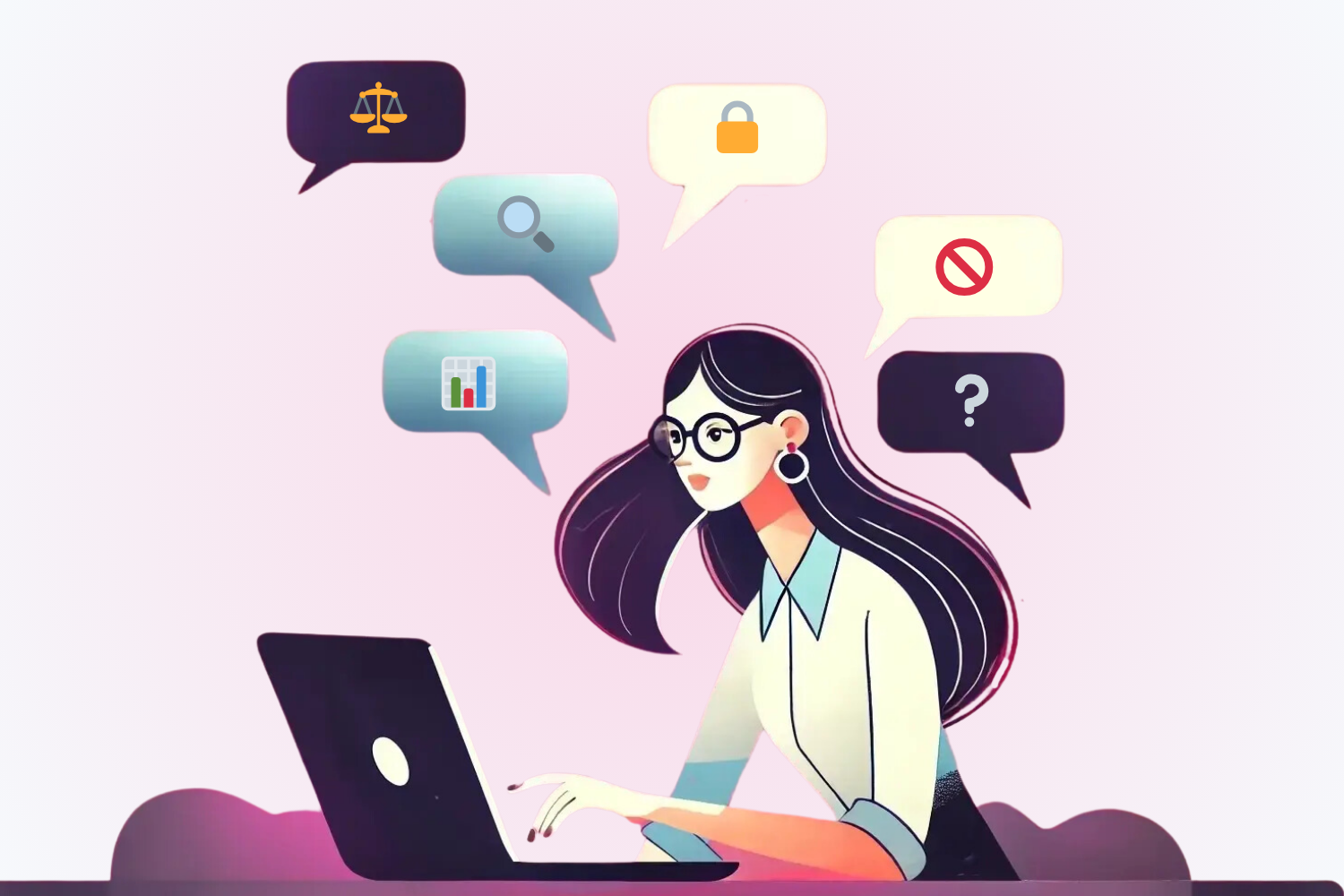
6 Common Myths About AI, Insurance, and Compliance
I run into the same misconceptions about AI and insurance again and again. Let me try to put some of these common myths to bed once and for all.
These days, my professional life is dedicated to one focused part of the global business landscape: the untamed frontier where cutting-edge AI meets insurance.
I have conversations with insurers around the world about where it’s all going and how AI will work under new global regulations. And one thing never ceases to amaze me: how often I end up addressing the same misconceptions.
Some confusion is understandable (if not inevitable) considering the speed with which these technologies are evolving, the hype from those suddenly wanting a piece of the action, and some fear-mongering from an old guard seeking to maintain the status quo. So, I thought I’d take a moment to clear the air and address six all-too-common myths about AI in insurance.
Myth 1: You’re not allowed to use AI in insurance
Yes, there’s a patchwork of emerging AI regulations—and, yes, in many cases they do zero-in specifically on insurance—but they do not ban its use. From my perspective, they do just the opposite: They set ground rules, which frees carriers to invest in innovation without fear they are developing in the wrong direction and will be forced into a hard pivot down the line.
Sixfold has actually increased customers (by a lot) since the major AI regulations in Europe and elsewhere were announced. So, let’s put this all-too-prevalent misconception to bed once and for all. There are no rules prohibiting you from implementing AI into your insurance processes.
Myth 2: AI solutions can’t secure customer data
As stated above, there are no blanket prohibitions on using customer data in AI systems. There are, however, strict rules dictating how data—particularly PII and PHI—must be managed and secured. These guidelines aren’t anything radically new to developers with experience in highly regulated industries.
Security-first data processes have been the norm since long before LLMs went mainstream. These protocols protect crucial personal data in applications that individuals and businesses use every day without issue (digital patient portals, browser-based personal banking, and market trading apps, just to name a few). These same measures can be seamlessly extended into AI-based solutions.
Myth 3: “My proprietary data will train other companies’ models”
No carrier would ever allow its proprietary data to train models used by competitors. Fortunately, implementing an LLM-powered solution does not mean giving up control of your data—at least with the right approach.
A responsible AI vendor helps their clients build AI solutions trained on their unique data for their exclusive use, as opposed to a generic insurance-focused LLM to be used by all comers. This also means allowing companies to maintain full control over their submissions within their environment so that when, for example, a case is deleted, all associated artifacts and data are removed across all databases.
At Sixfold, we train our base models on public and synthetic (AKA, “not customer”) data. We then copy these base models into dedicated environments for our customers and all subsequent training and tuning happens in the dedicated environments. Customer guidelines and data never leave the dedicated environment and never make it back to the base models.
Let’s kill this one: Yes, you can use AI and still maintain control of your data.
Myth 4: There’s no way to prevent LLM hallucinations
We’ve all seen the surreal AI-generated images lurching up from the depths of the uncanny valley—hands with too many fingers, physiology-defying facial expressions, body parts & objects melded together seemingly at random. Surely, we can’t use that technology for consequential areas like insurance. But I’m here to tell you that with the proper precautions and infrastructure, the impact of hallucinations can be greatly minimized, if not eliminated.
Mitigation is achieved using a myriad of tactics such as using models to auto-review generated content, incorporating user feedback to identify and correct hallucinations, and conducting manual reviews to ensure quality by comparing sample outputs against ground truth sets.
Myth 5: AIs run autonomously without human oversight
Even if you never watched The Terminator, The Matrix, 2001: A Space Odyssey, or any other movie about human-usurping tech, it’d be reasonable to have some reservations about scaled automation. There’s a lot of fearful talk out there about humans ceding control in important areas to un-feeling machines. However, that’s not where we’re at, nor is it how I see these technologies developing.
Let’s break this one down.
AI is a fantastic and transformative technology, but even I—the number one cheerleader for AI-powered insurance—agree we shouldn’t leave technology alone to make consequential decisions like who gets approved for insurance and at what price. But even if I didn’t feel this way, insurtechs are obliged to comply with new regulations (e.g., the EU AI Act and the California Department of Insurance), that tilt towards avoiding fully automated underwriting and require, at the very least, that humans overseers can audit and review decisions.
When it comes to your customers’ experience, AI opens the door to more human engagement, not less. In my view, AI will free underwriters from banal, repetitive data work (which machines handle better anyway) so that they can apply uniquely human skills in specialized or complex use cases they previously wouldn’t have had the bandwidth to address.
Myth 6: Regulations are still being written, it’s better to wait for them to settle
I hear this one a lot. I understand why people arrive at this view. My take? You can’t afford to sit on the sidelines!
To be sure, multiple sets of AI regulations are taking root at different governmental levels, which adds complexity. But here’s a little secret from someone paying very close attention to emerging AI rulesets: there’s very little daylight between them.
Here’s the thing: regulators worldwide attend the same conferences, engage with the same stakeholders, and read the same studies & whitepapers. And they all watching what each other is doing. As a result, we’re arriving at a global consensus focused on three main areas: data security, transparency, and auditability.
The global AI regulatory landscape is, like any global regulatory landscape, complex; but I’m here to tell you it’s not nearly as uneven or even close to unmanageable as you may fear.
Furthermore, if an additional major change were to be introduced, it wouldn't suddenly take effect. That’s by design. Think of all the websites and digital applications that launched—and indeed, thrived—in the six-year window between when GDPR was introduced in 2012 to when it became enforceable in 2018. Think of everything that would have been lost if they had waited until GDPR was firmly established before moving forward.
My entire career has been spent in fast-moving cutting-edge technologies. And I can tell you from experience that it’s far better to deploy & iterate than to wait for regulatory Godot to arrive. Jump in and get started!
There are more myths to bust! Watch our compliance webinar
The regulations coming are not as odious or as unmanageable as you might fear—particularly when you work with the right partners. I hope I’ve helped overcome some misconceptions as you move forward on your AI journey.
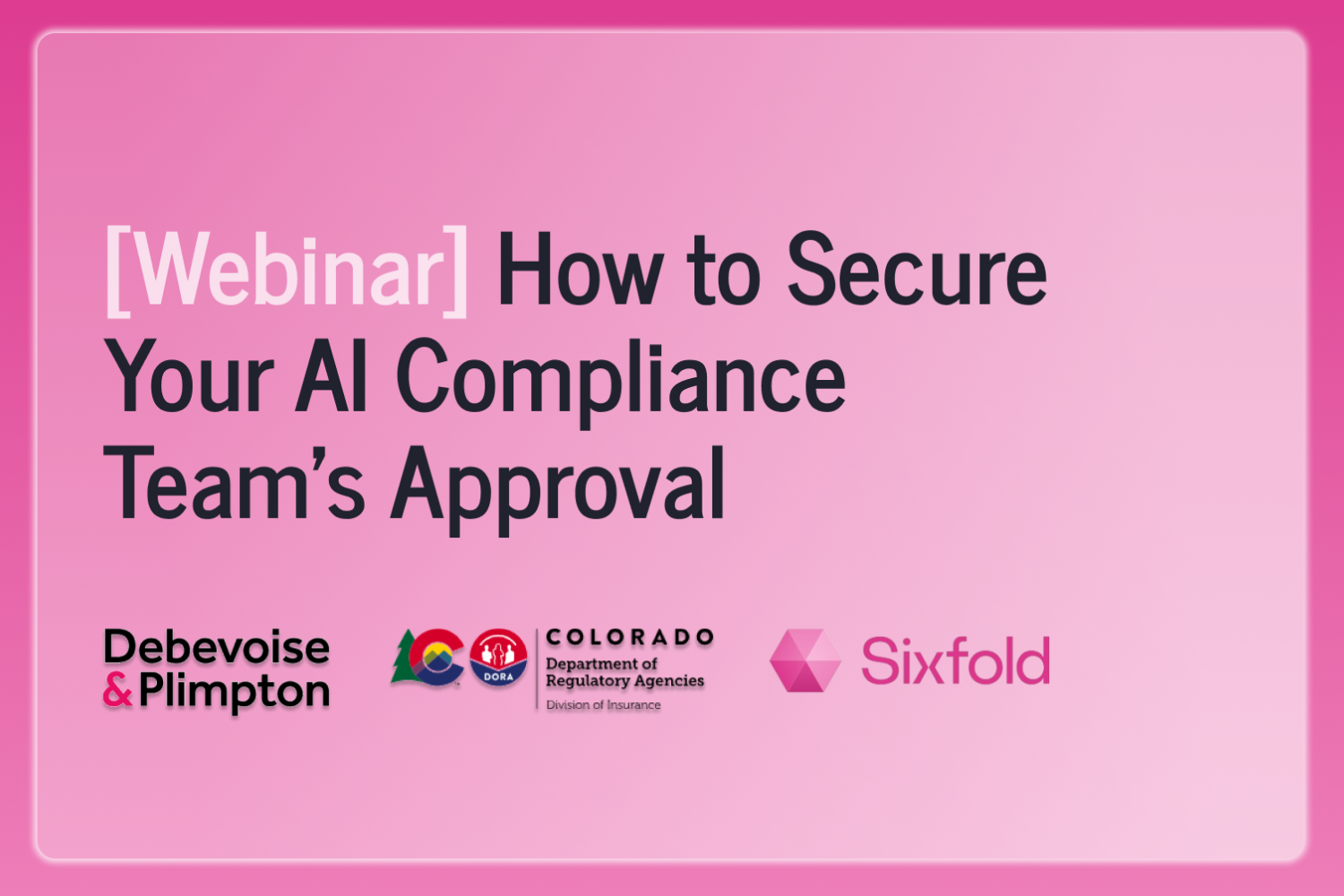
Want to learn more about AI insurance and compliance? Watch the replay of our compliance webinar featuring a discussion between myself; Jason D. Lapham, the Deputy Commissioner for P&C Insurance the Colorado Division of Insurance; and Matt Kelly a key member of Debevoise & Plimpton’s Artificial Intelligence Group. We're discussing the global regulatory landscape and how AI models should be evaluated regarding compliance, data usage, and privacy.
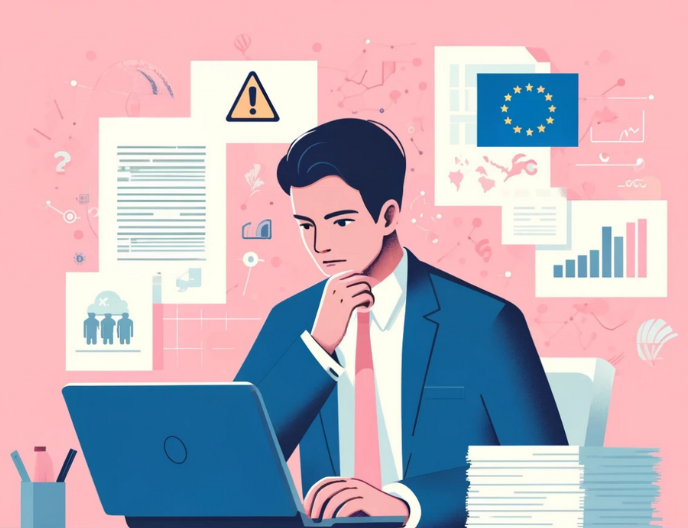
AI in Insurance Is Officially “High Risk” in the EU. Now What?
The new EU AI Act defines AI in insurance as “high risk.” Here’s what that means and how to remain compliant in Europe and around the world.
The European Parliament passed the EU Artificial Intelligence Act in March, a sweeping regulatory framework scheduled to go into effect by mid-2026.
The Act categorizes AI systems into four risk tiers—Unacceptable, High, Limited, and Minimal—based on the sensitivity of the data the systems handle and the crucialness of the use case.
It specifically carves out guidelines for AI in insurance, placing “AI systems intended to be used for risk assessment and pricing in [...] life and health insurance” in the “High-risk” tier, which means they must continually satisfy specific conditions around security, transparency, auditability, and human oversight.
The Act’s passage is reflective of an emerging acknowledgment that AI must be paired with rules guiding its impact and development—and it's far from just an EU thing. Last week, the UK and the US signed a first-of-its-kind bilateral agreement to develop “robust” methods for evaluating the safety of AI tools and the systems that underpin them.
I fully expect to see additional frameworks following the EU, UK, and US’s lead, particularly within vital sectors such as life insurance. Safety, governance, and transparency are no longer lofty, optional aspirations for AI providers, they are inherent—and increasingly enforceable—facets of the emerging business landscape.
Please be skeptical of your tech vendors
When a carrier integrates a vendor into their tech stack, they’re outsourcing a certain amount of risk management to that vendor. That’s no small responsibility and one we at Sixfold take very seriously.
We’ve taken on the continuous work of keeping our technology compliant with evolving rules and expectations, so you don’t have to. That message, I’ve found, doesn’t always land immediately. Tech leaders have an inherent “filter” for vendor claims that is appropriate and understandable (I too have years of experience overseeing sprawling enterprise tech stacks and attempting to separate marketing from “the meat”). We expect—indeed, we want—customers to question our claims and check our work. As my co-founder and COO Jane Tran put it during a panel discussion at ITI EU 2024:
“As a carrier, you should be skeptical towards new technology solutions. Our work as a vendor is to make you confident that we have thought about all the risks for you already.”
Today, confidence-building has extended to ensuring customers and partners that our platform complies with emerging AI rules around the world—including ones that are still being written.
Balancing AI underwriting and transparency
When we launched last year, there was lots of buzz about the potential of AI, along with lots of talk about its potential downside. We didn’t need to hire pricey consultants to know that AI regulations would be coming soon.
Early on, we actively engaged with US regulators to understand their thinking and offer our insights to them as AI experts. From these conversations, we learned that the chief issue was the scaling out of bias and the impact of AI hallucinations on consequential decisions.

With these concerns in mind, we proactively designed our platform with baked-in transparency to mitigate the influence of human bias, while also installing mechanisms to eliminate hallucinations and elevate privacy. Each Sixfold customer operates within an isolated, single-tenant environment, and end-user data is never persisted in the LLM-powered Gen AI layer so information remains protected and secure. We were implementing enterprise AI guardrails before it was cool.
I’ve often found customers and prospects are surprised when I share with them how prepared our platform is for the evolving patchwork of global AI regulations. I’m not sure what their conversations with other companies are like, but I sense the relief when they learn how Sixfold was built from the get-go to comply with the new way of things–even before they were a thing.
The regulatory landscape for AI in insurance is developing quickly, both in the US and globally. Join a discussion with industry experts and learn how to safely and compliantly integrate your next solution. Register for our upcoming webinar here >
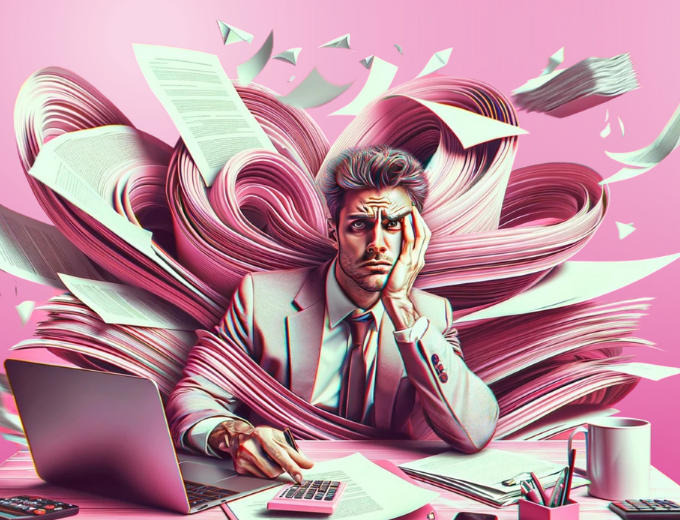
It Takes You Months to Train AIs? You're Doing It Wrong
Discover the power of AI underwriting with Sixfold - the ultimate solution to overcome traditional limits and stay ahead in the insurance industry.
It’s impossible in 2024 to be an insurance carrier and not also be an AI company. In this most data-focused of sectors, the winners will be the organizations making the best use of emerging AI tech to amplify capacity and improve accuracy.
This is a challenge and opportunity that Sixfold is uniquely suited to address thanks to our decades of collective industry and technological experience. We know insurers’ needs—intimately—and understand precisely how AI can overcome them.
In previous posts, I’ve described how Sixfold uses state-of-the-art AI to ingest data from disparate sources, surface relevant information, and generate plain-language summarizations. Our platform, in effect, provides every underwriter with a virtual team of researchers and analysts who know exactly what’s needed to render a decision. But getting there is the rub. Training AI models (these “virtual teams”) to understand what information is relevant for specific product lines is no small task, but it’s where Sixfold excels.
To use AI is human, to create your own unique AI model is divine
Underwriting guidelines aren’t typically encapsulated in a single machine-readable document. They’re more likely to exist in an unordered web of internal documents and reflected in historic underwriting decisions. Distilling a diffuse cultural understanding into an AI model can take months using a traditional approach, but with Sixfold, it can be accomplished—and accomplished well—in as little as a few days.
Sixfold’s proprietary AI captures carriers’ unique risk appetite by ingesting a wide variety of inputs (be it a multi-hundred-page PDF of guidelines, a loose assortment of spreadsheets, or even past underwriting decisions) and translating it into an AI model that knows what information aligns with a positive risk signal, a negative one, or a disqualifying factor.
With this virtual wisdom model in place, the platform can identify and ingest relevant data from submitted documents, supplement with information from public and third-party data sources, and generate semantic summaries of factors supporting its conclusions—all adhering to the carriers’ unique underwriting approach.
Frees human underwriters to do uniquely human tasks
It can take years for a human underwriter to master underwriting guidelines and rules, but that doesn’t mean human underwriters are no longer needed–quite the opposite. By offloading the administrative bulk to AI, underwriters can use their increased capacity to prioritize cases that align with their unique risk appetite.
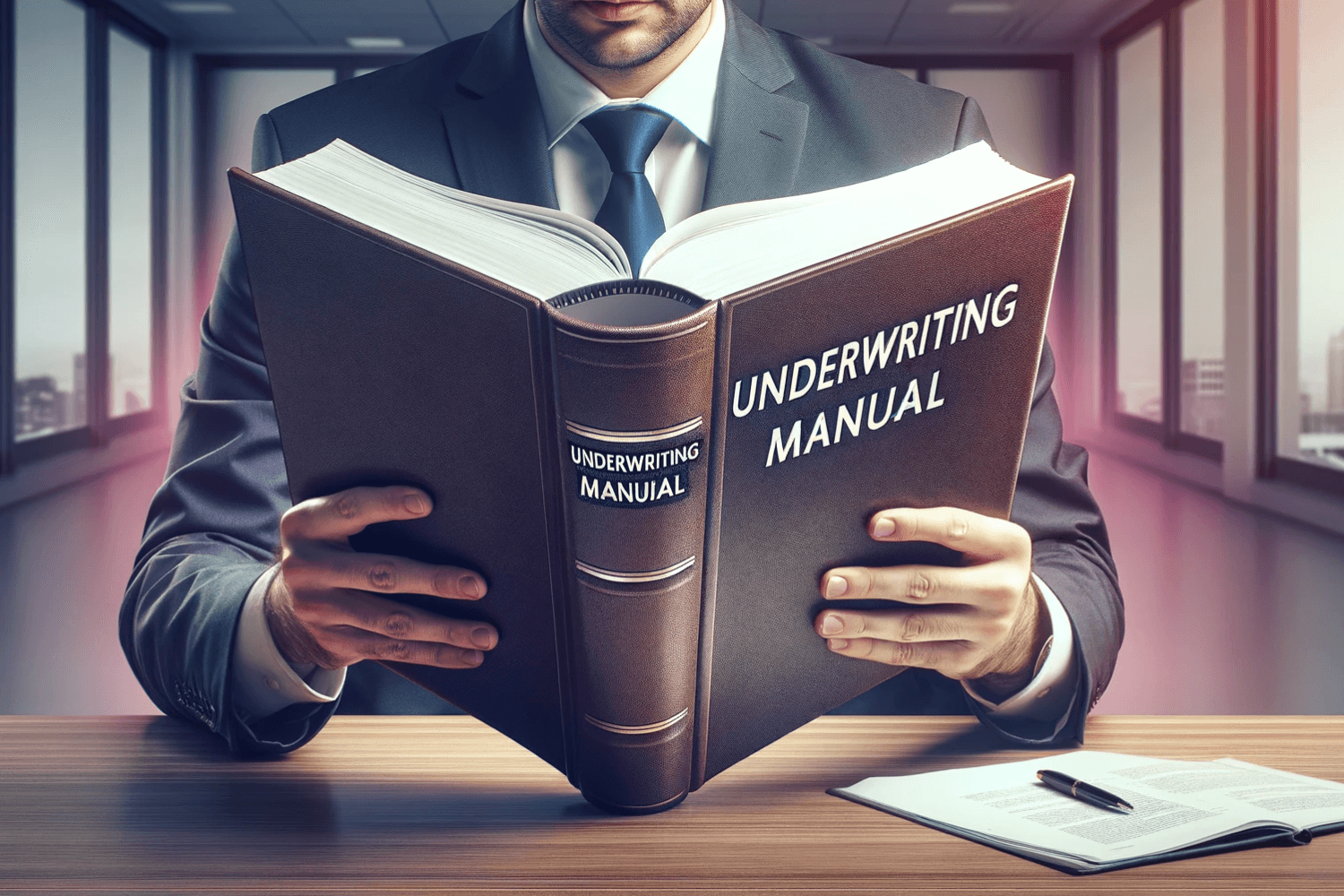
Consider a P&C carrier that prefers not to underwrite businesses that work with asbestos. When an application comes in, Sixfold’s platform processes all broker-submitted documents and supplements it with relevant data ingested from public and third-party data sources. If Sixfold were to then surface information about “assistance with obtaining asbestos abatement permits” from the applicant’s company website, it would automatically mark the finding as a negative risk signal (with clear sourcing and semantic explanation) in the underwriter-facing case dashboard. With Sixfold, underwriters can rapidly discern the applications that are incompatible with their underwriting criteria and quickly focus on cases aligned with their risk appetite.
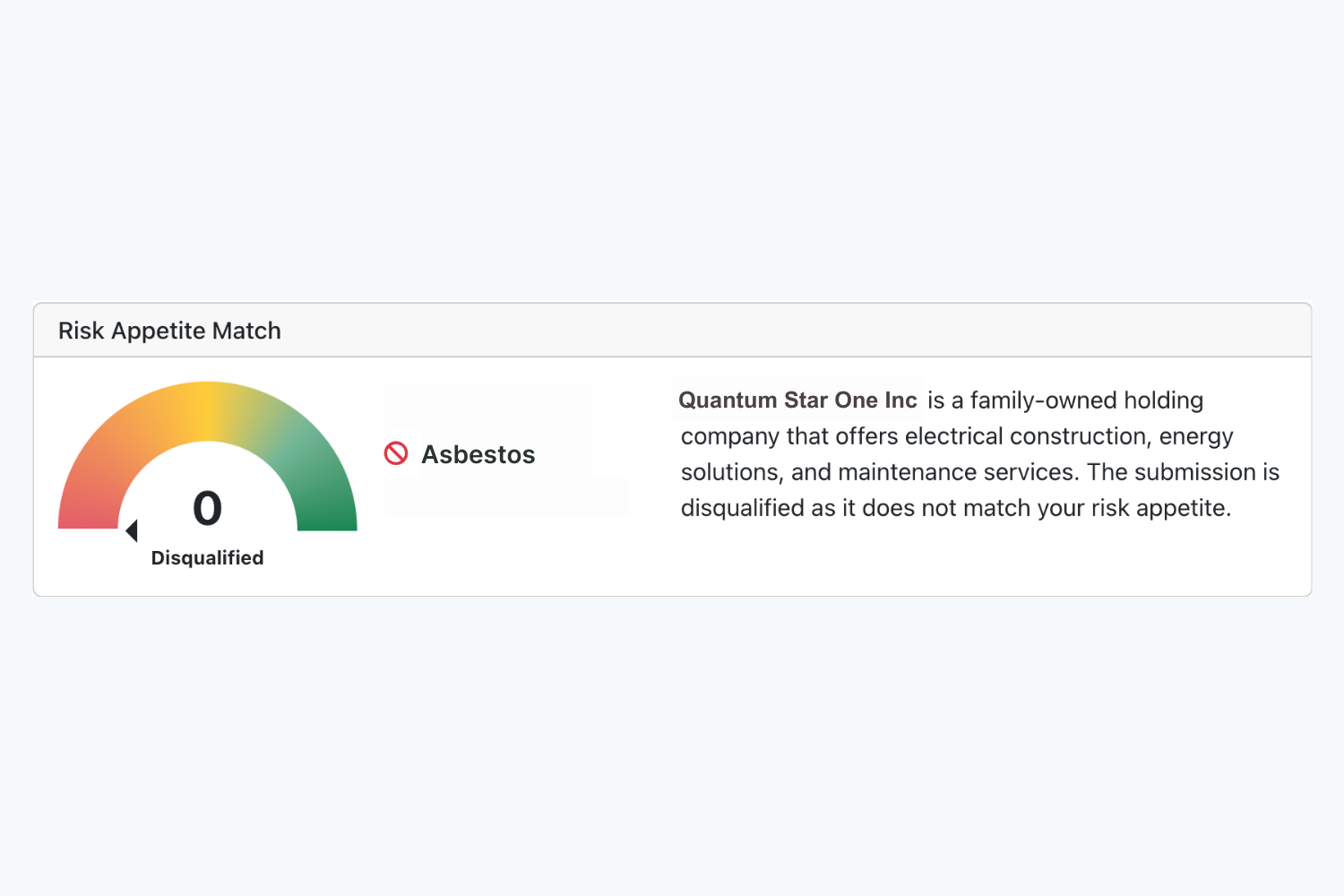
Automating these previously resource-intensive data processing workflows allows carriers to obliterate traditional limits on underwriting capacity. The question for the industry has rapidly moved from “is automation possible?” to “how quickly can we get it implemented?” Sixfold’s purpose-built platform empowers customers to leapfrog competitors relying on traditional approaches to training AI underwriting models. We get you there faster–this is our superpower.

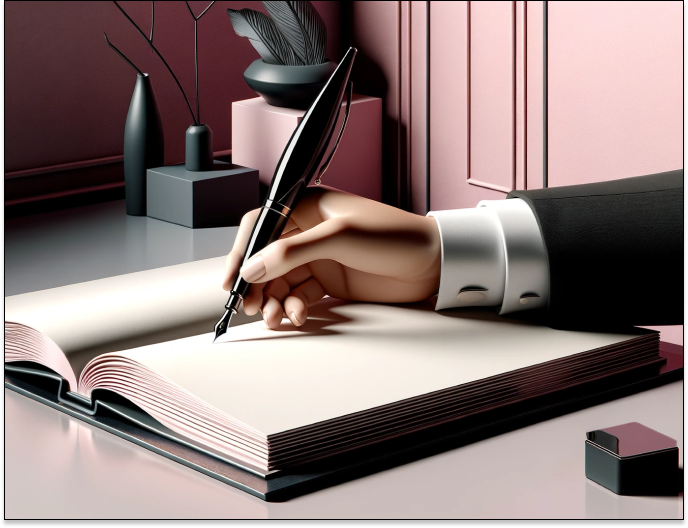
Navigating Unwritten Regulations: How We Did It
As regulators at all levels of government are drafting rules guiding the usage of artificial intelligence, Sixfold AI built a platform for unwritten rules.
AI is the defining technology of this decade. After years of unfulfilled promises from Hollywood and comic books, the science fiction AI future we’ve long been promised has finally become business reality.
We can already see AI following a familiar path through the marketplace similar to past disruptive technologies.
- Stage one: it’s embraced by early adopters before the general public even knows it exists;
- Stage two: cutting-edge startups tap these technologies to overcome long-standing business challenges; and then
- Stage three: regulators draft rules to guide its usage and mitigate negative impacts.
There should be no doubt that AI-powered insurtech has accelerated through the first two stages in near record time and is now entering stage three.
AI underwriting solutions, meet the rule-makers
The Colorado Department of Regulatory Agencies recently adopted regulations on AI applications and governance in life insurance. To be clear, Colorado isn’t an outlier, it’s a pioneer. Other states are following suit and crafting their own AI regulations, with federal-level AI rules beginning to take shape as well.
The early days of the regulatory phase can be tricky for businesses. Insurers are excited to adopt advanced AI into their underwriting tech stack, but wary of investing in platforms knowing that future rules may impact those investments.
We at Sixfold are very cognizant of this dichotomy: The ambition to innovate ahead, combined with the trepidation of going too far down the wrong path. That’s why we designed our platform in anticipation of these emerging rules.
We’ve met with state-level regulators on numerous occasions over the past year to understand their concerns and thought processes. These engagements have been invaluable for all parties as their input played a major role in guiding our platform’s development, while our technical insights influenced the formation of these emerging rules.
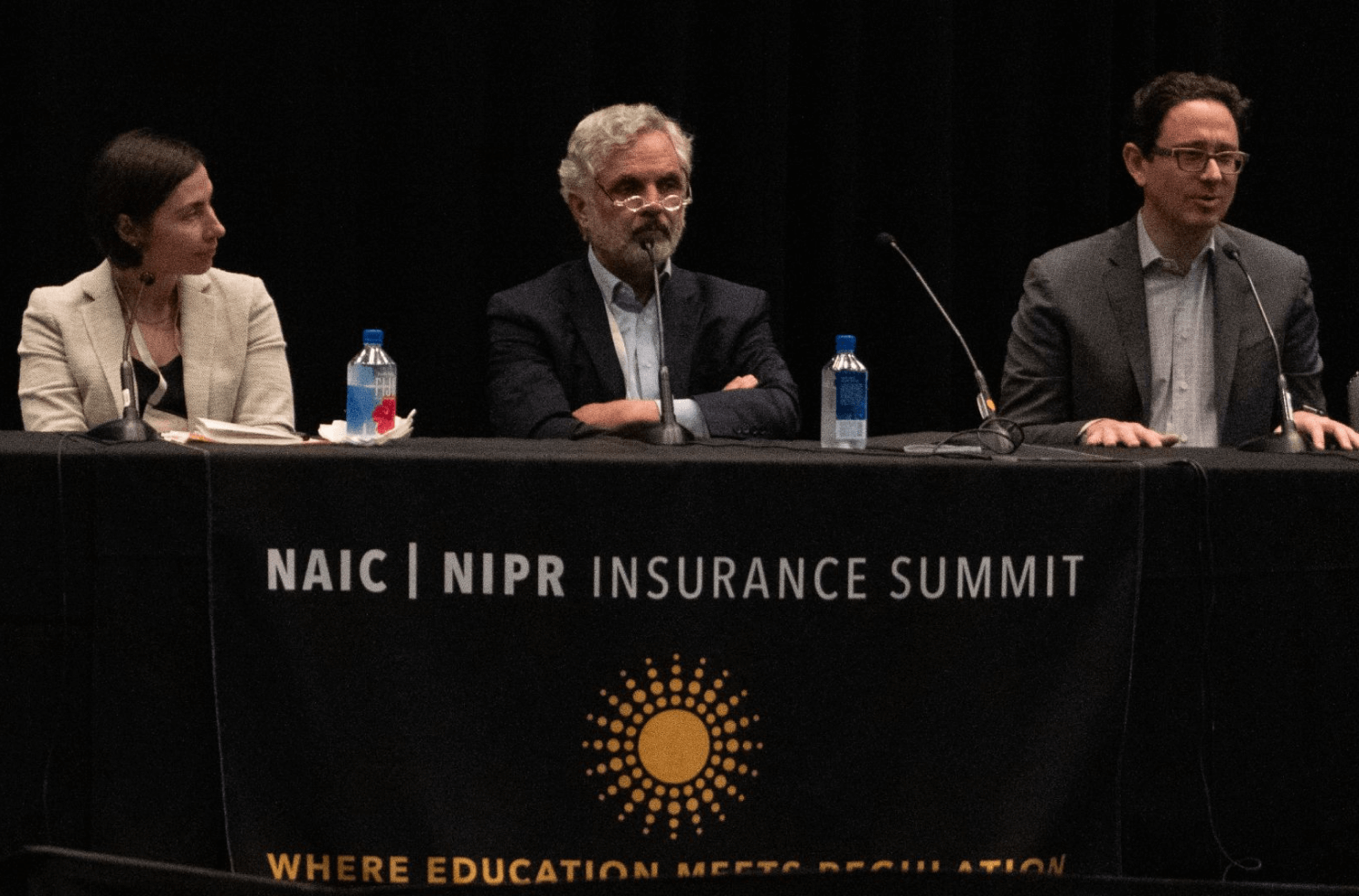
To simplify a very complex motion: regulators are concerned with bias in algorithms. There’s a tacit understanding that humans have inherent biases, which may be reflected in algorithms and applied at scale.
Most regulators we’ve engaged with agree that these very legitimate concerns about bias aren’t a reason to prohibit or even severely restrain AI, which brings enormous positives like accelerated underwriting cycles, reduced overhead, and increased objectivity–all of which ultimately benefit consumers. However, for AI to work for everyone, it must be partnered with transparency, traceability, and privacy. This is a message we at Sixfold have taken to heart.
In AI, it’s all about transparency
The past decade saw a plethora of algorithmic underwriting solutions with varying degrees of capabilities. Too often, these tools are “black boxes” that leave underwriters, brokers, and carriers unable to explain how decisions were arrived at. Opaque decision-making no longer meets the expectations of today’s consumers—or of regulators. That’s why we designed Sixfold with transparency at its core.
Customers accept automation as part of the modern digital landscape, but that acceptance comes with expectations. Our platform automatically surfaces relevant data points impacting its recommendations and presents them to underwriters via AI-generated plain-language summarizations, while carefully controlling for “hallucinations.” It provides full traceability of all inputs, as well as a full lineage of changes to the UW model, so carriers can explain why results diverged over time. These baked-in layers of transparency allow carriers–and the regulators overseeing them–to identify and mitigate incidental biases seeping into UW models.
Beyond prioritizing transparency, we‘ve designed a platform that elevates data security and privacy. All Sixfold customers operate within isolated, single-tenant environments, and end-user data is never persisted in the LLM-powered Gen AI layer so information remains protected and secure.
Even with platform features built in anticipation of external regulations, we understand that some internal compliance teams are cautious about integrating gen AI, a relatively new concept, into their tech stack. To help your internal stakeholders get there, Sixfold can be implemented with robust internal auditability and appropriate levels of human-in-the-loop-ness to ensure that every team is comfortable on the new technological frontier.
Want to learn more about how Sixfold works? Get in touch.

We at Sixfold believe regulators play a vital role in the marketplace by setting ground rules that protect consumers. As we see it, it’s not the technologist’s place to oppose or confront regulators; it’s to work together to ensure that technology works for everyone.
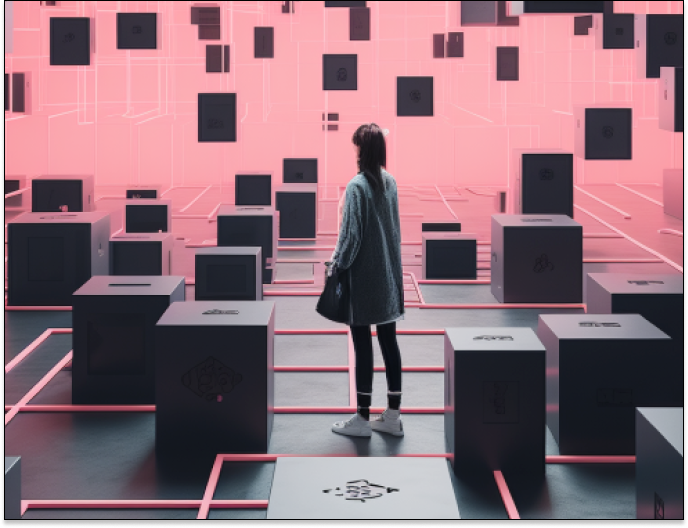
The Unspoken Truth: InsurTech AI's Struggle to Keep Up
Sixfold's state-of-the-art insurtech AI revolutionizes the insurance industry, tackling today's challenges head-on and preparing for the future.
The past decade saw more than its fair share of insurtech solutions promising to harness the power of “AI.” Many of these tools use hard-to-train algorithms powered by technologies that are years—if not decades—old. These legacy underwriting tools may inject some process efficiencies but don’t address the fact that insurers are struggling more than ever to expand capacity and grow Gross Written Premiums (GWPs) per underwriter.
Underwriters face a lot of issues
A recent Accenture survey found that underwriters spend 40% of their time on administrative tasks—that’s a full two days of their work week. Inboxes are flooded with more submissions than ever, but by some estimates, underwriters are only able to respond to 10%.
These aren’t challenges that companies can simply spend their way around; they require a fundamentally new approach. At Sixfold, we believe ascendent technologies, like LLM-powered generative AI, will lead the way. By moving beyond legacy solutions, carriers can take on today’s most pressing underwriting challenges–and the challenges on the horizon.
Ingesting and synthesizing data from disparate sources at scale
In the connected-everything world, insurers have access to more data than ever. This is a blessing and a challenge. On one hand, it guides decisioning and improves outcomes. On the other hand, there’s so much data from so many disparate sources, that it’s impossible to process efficiently.
Underwriters often find themselves sorting through hundreds of pages of documents for a single application. This limits capacity and squeezes GWP per underwriter. The only way to overcome these chokepoints without massively expanding headcount (and dinging already precarious expense ratios) is by using sophisticated AI tools to automate complex business tasks at scale.
With Sixfold’s state-of-the-art underwriting AI, insurers can seamlessly integrate structured and unstructured data from multiple disparate sources. The platform reflects each company's unique risk appetite, so it automatically surfaces relevant information to accelerate UW decisioning.
Say it in plain language
Sixfold uses LLM-powered generative AI (the same tech behind ChatGPT, Bard, etc.) to summarize findings to underwriters in plain language, not spreadsheets.
The platform, in effect, gives every underwriter their own virtual research team to build detailed reports on every application. Sixfold even generates coverage recommendations based on the company’s UW format. Compare this to legacy AI tools, which merely repackage information into number-heavy spreadsheets and dashboards, inevitably requiring additional inspection and contextualization from underwriters.
Even better? Plain language summations expand the underwriting talent pool by de-emphasizing technical and computational skillsets that are better handled by machines anyway. This is a crucial break from legacy tools, as insurers are now forced to compete for limited underwriting talent against private-equity-backed firms, insurtechs, MGAs, and other nontraditional insurance companies.
Opacity in insurance is no longer an option, AI transparency is the new norm
Sixfold was designed with transparency at its core because that’s what today’s customers expect and increasingly what regulators demand. The platform provides full sourcing and lineage of all underwriting decisions with clear semantic summaries, i.e. no more “black boxes.”
.png)
Customers accept automation as part of the modern digital landscape, but that acceptance comes with expectations of transparency, particularly when there are unexpected outcomes. Legacy solutions make it difficult—if not impossible—for insurers to provide customers with the clarity they deserve. Disappointed customers and diminished brand reputation, however, aren’t the only negative outcomes the industry needs to be mindful of.
As scaled automation becomes more ubiquitous, so have the calls for greater transparency from . At all levels of government, there are movements to counter the influence of potential bias through increased transparency and accountability—particularly in crucial areas like insurance.
The marketplace has long since moved on from “because the algorithm said so,” and insurers must employ tools to reflect those changes.
Beyond legacy AI
We’re not the first to automate underwriting tasks using “AI,” but we’re the first to fundamentally reimagine the underwriting role using state-of-the-art LLM tech to generate business value. Customers are using our platform to accelerate submission-to-quote cycles by as much as 43% and massively increase their GWP per underwriter.
The role of the underwriter is evolving, and the industry needs a new generation of tools to match. This is why we created Sixfold.