Sixfold Content
Product Update
Meet the First AI Accuracy Validator Built for Insurance Underwriting
Today, we’re excited to introduce the first-ever AI Accuracy Validator built for insurance underwriting. This application provides customers with a transparent and comprehensive way to evaluate Sixfold’s accuracy—reinforcing our commitment to bring reliable and trustworthy risk assessments to underwriters.

Explore all Resources
Stay informed, gain insights, and elevate your understanding of AI's role in the insurance industry with our comprehensive collection of articles, guides, and more.
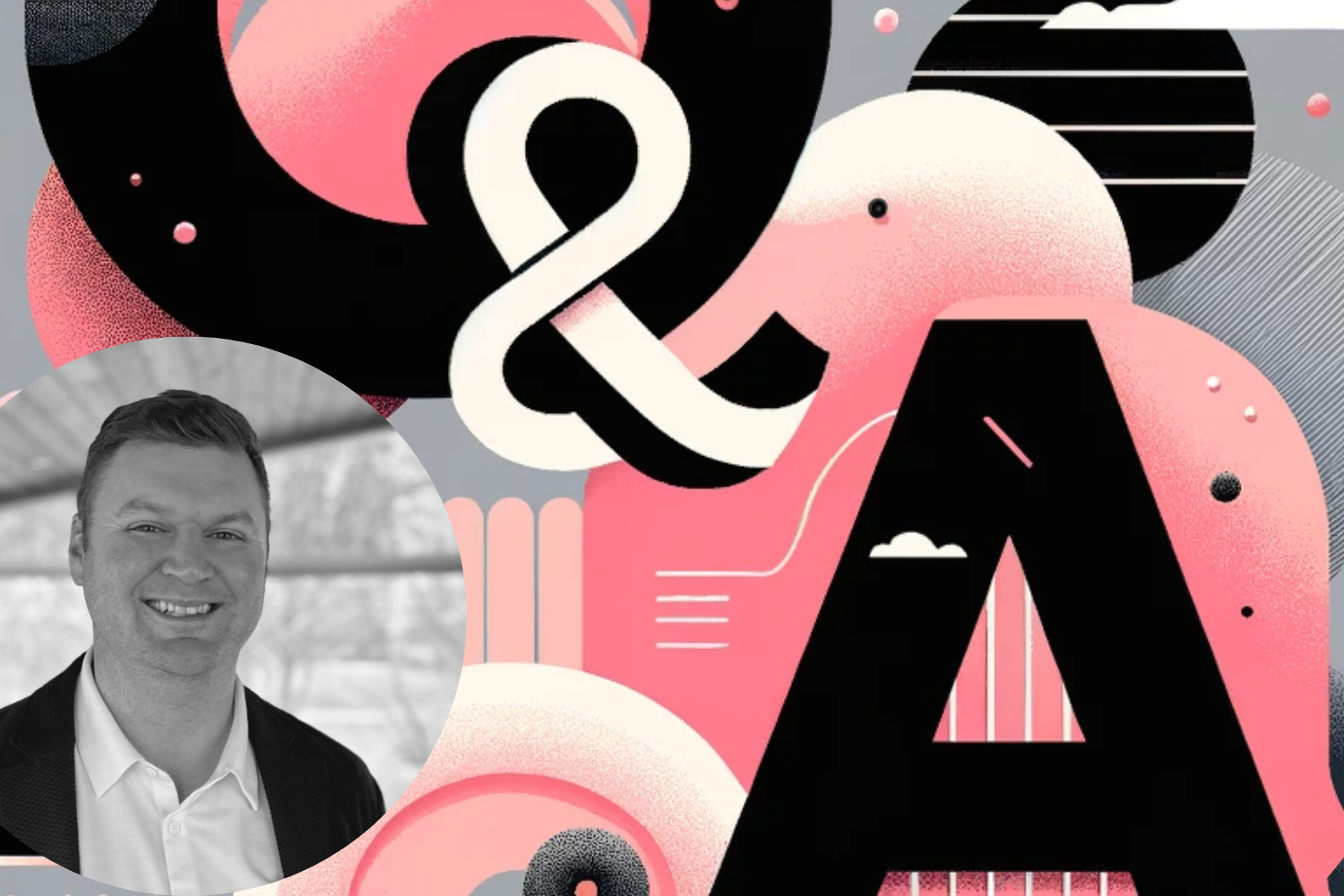
Q&A with Adam Nunes, Sixfold’s New Sales Director
Adam Nunes recently joined Sixfold as our new Sales Director. In this Q&A, he talks about growing up in a family insurance business, the changes he’s seen in the industry, and why he’s excited to help carriers adopt AI that actually works for underwriters.
How long have you been working in the insurance and insurtech industry? Tell us about that!
There’s an old adage in the insurance world: you were either born into insurance, or you tripped and fell into it. I’m firmly in the former category! Insurance has always been the family business, and I started my career quite literally at the footstool of our family agency.
I began on the distribution side of the business, working as a producer, but over time, I realized the value I could bring to insurers by being a part of the technological change for good.
And after being part of the insurance ecosystem for so long, I honestly couldn’t imagine doing anything else.
What are some of the biggest changes you’ve witnessed during your time in the insurance space?
The easy answer is to say “AI” and “Machine Learning.” And yes, those are monumental advancements that are already transforming how carriers operate. But they won’t change the core business of insurance.
To me, the bigger shift has been the role the business now plays in technology decisions. With so many new tools focused on solving business problems, underwriting leaders need to be active stakeholders in technology decisions, rather than passive consumers.
To me, the bigger shift has been the role the business now plays in technology decisions. With so many new tools focused on solving business problems, underwriting leaders need to be active stakeholders in technology decisions, rather than passive consumers.
There’s an overwhelming number of options and decisions to be made to modernize underwriting. That’s why it’s more important than ever for the business to drive decisions and to collaborate closely with their partners in IT.
What was the first product or service you ever sold?
That’s an easy one—insurance! I’ve been around the industry since before I could walk, and it was the very first product I learned to sell.
What’s unique about selling insurance is that it’s not a tangible good. You really have to understand someone’s business and their risks before you can make the right coverage or policy recommendation.
That mindset of trying to understand before making a recommendation has been something I’ve carried with me throughout my career. At the end of the day, technology is only as valuable as the business problem it solves.
With underwriting technology evolving rapidly, how do you approach understanding and anticipating the needs of customers in such a fast-moving space?
Anticipating a customer’s tech needs starts with understanding their business. I’m an insurance person first and, a technologist second. And while technology continues to evolve at a rapid pace, the core business model of insurance hasn’t really changed.
The most important part of my job is understanding the nuances of how each insurer operates, so I can help guide them toward solutions that solve their unique business needs, while also taking into account where they are today.
How do you stay on top of the latest industry trends?
I’m a voracious reader of insurance content and trends, but honestly, my greatest learnings come from the friends I’ve made along the way, the peers I respect deeply, and the clients I work with.
One of the beautiful parts about the insurance community is that there’s always something new to learn and people who are willing to share their knowledge. It’s amazing how helpful folks are when you’re genuinely trying to learn.
Looking back at your career, what are some key lessons you've learned that you plan to bring to your role at Sixfold?
There are two main lessons I’ve learned along my career. The first is that insurance has been and always will be a people business. Even though insurance companies are large, complex organizations, they’re made up of incredible individuals who genuinely want the industry to be a force for good.
The second lesson is incredibly important: selling software can be challenging–but buying software for a large, complex organization is even harder. That’s especially true when the technology is new and evolving quickly. It’s important for us to remember that in every interaction.
The second lesson is incredibly important: selling software can be challenging–but buying software for a large, complex organization is even harder. That’s especially true when the technology is new and evolving quickly. It’s important for us to remember that in every interaction.
That’s why I don’t see my mission as “selling” software, but as helping insurers make an informed, consensus decision about the best options available to them.
What do you think sets Sixfold apart in this space and what excites you the most about joining the team?
While Sixfold is the first generative AI solution built specifically for insurance underwriting, what really excites me is that Sixfold is solving problems that have challenged insurers for years. Whether by solving the front door problem for P&C carriers or bringing the right medical context to life & disability underwriters, Sixfold addresses real-life business problems.
We meet underwriters where they are—we don’t ask them to change how or where they work.
Besides, insurers today face IT resource constraints, legacy systems, and never-ending implementation cycles. Sixfold inverts the paradigm. We meet underwriters where they are—we don’t ask them to change how or where they work. We prioritize explainability, and we don’t need an army of tech resources to get up and running quickly.
Want to join Adam and the rest of the Sixfold team? Check out our job openings here.
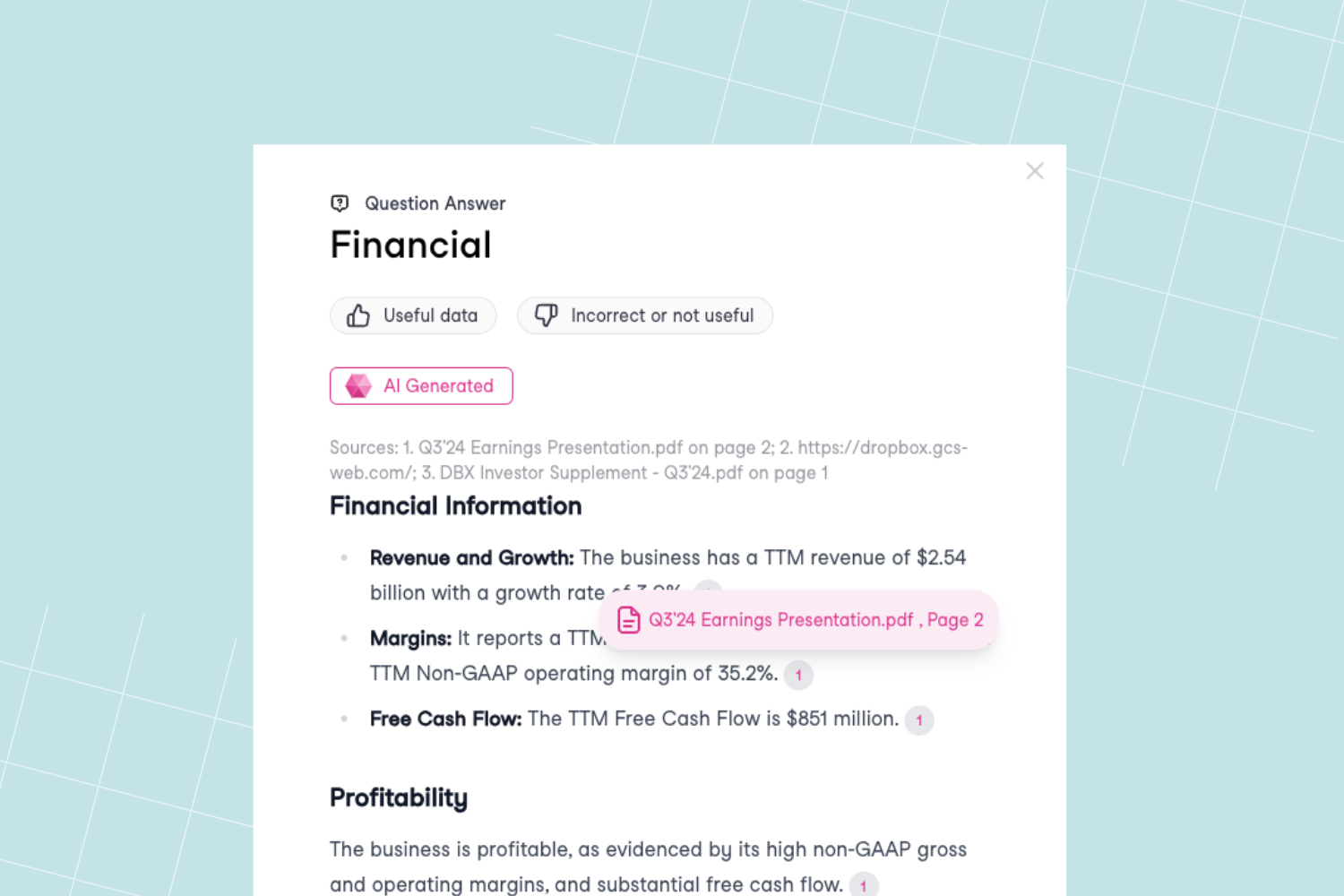
Now Live: One-Click Source Verification for Every Fact
Sixfold’s in-line citation feature is all about building trust and confidence with underwriters by making it effortless to trace the source of Sixfold’s insights. And now, with our latest update we’re making this feature even better!
Sixfold’s in-line citation feature is all about building trust and confidence with underwriters by making it effortless to trace the source of Sixfold’s insights. And now, with our latest update we’re making this feature even better on our commercial platform!
So, what is new?
When reviewing an applicant’s case, you'll notice these improvements:
- Exact Source Page: No more searching through the entire document—now you’ll see the exact page where a fact was found.
- In-line Fact Attribution: Each fact provided by Sixfold will include a specific source citation.
- One-Click Verification: Click any citation, and it will instantly open the source page for you.
It’s now even easier and more intuitive to check exactly where Sixfold’s insights came from—whether a questionnaire, loss run statement, webpage, or any other source. This improves traceability and gives underwriters extra peace of mind. No need to read through a full report or scroll through multiple pages to validate Sixfold’s insights.
“This feature exemplifies our commitment to building trustworthy and explainable AI with elegant simplicity.”
- Drew Das, AI Software Engineer
Get in touch to get a full demo of Sixfold's platform.

The Role of AI and IDP in Underwriting’s Future
Underwriting faces a data challenge, with manual processes consuming up to 40% of underwriters’ time. While IDP tools digitize data, underwriting AI goes further—providing actionable insights and enabling smarter risk analysis to improve efficiency and accuracy.
Insurance underwriting has long struggled with a data challenge: finding a way to handle the daily flood of information quickly and accurately. Why? Because the ability to process data significantly impacts the profitability and growth of insurers.
If this sounds familiar, here’s some good news: there’s now a better way to tackle these challenges. Underwriting-focused AI is transforming underwriting by processing complex data, providing risk summaries, and delivering tailored risk recommendations—all in ways that were previously unimaginable.
Most of the information underwriters need to make an underwriting decision comes in a mix of structured, semi-structured, and unstructured formats.
Most of the information underwriters need to make an underwriting decision comes in a mix of structured, semi-structured, and unstructured formats, including various types of documents such as broker emails, application forms, and loss runs. This data is often handled manually, consuming 30% to 40% of underwriters' time and ultimately impacting Gross Written Premium (McKinsey & Company).
To address this, insurers have long sought technological solutions. Intelligent Document Processing (IDP) tools were a key step forward, using technologies like Optical Character Recognition (OCR) to extract and organize data. However, while IDP helps digitize information, it doesn’t fully solve the problem of turning data into actionable insights.
Comparing IDP and Underwriting AI
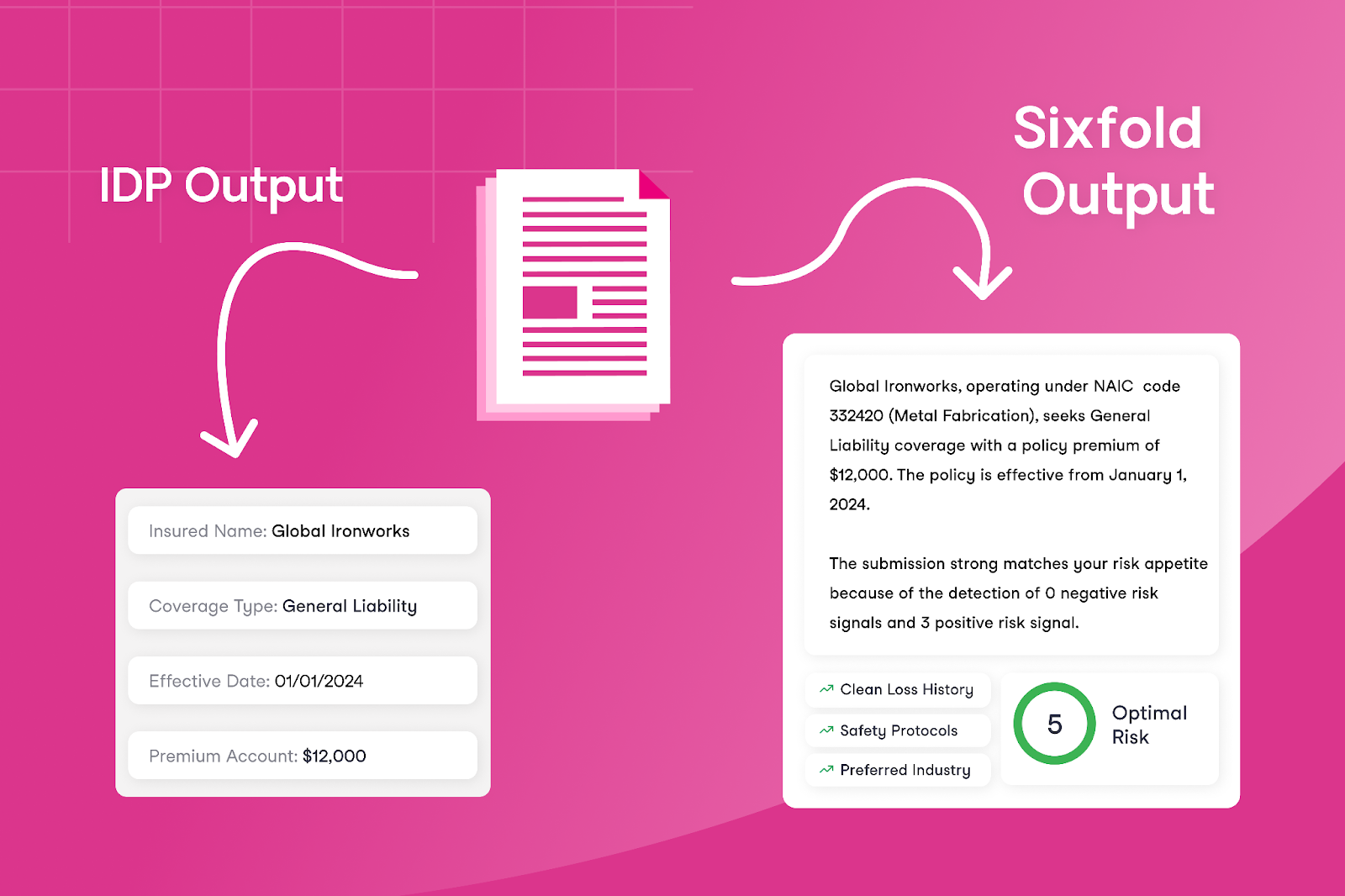
IDPs have been the predominant way of approaching underwriting efficiency, primarily used by insurers to automate tasks in underwriting, policy administration, and claims processing. These tools focus on converting generic documents into structured data by capturing text, classifying document types, and extracting key fields, offering a general solution across different industries.
Digitizing documents - sounds like a no-brainer right? But, what happens if you go beyond simply digitizing documents?
Digitizing documents - sounds like a no-brainer right? But, what happens if you go beyond simply digitizing documents? Underwriting AI makes this possible by offering a new approach to improving efficiency and accuracy for underwriters — by not just bringing in all the data but also generating risk analysis and actionable insights. AI empower underwriting teams to focus only on the information that truly matters for underwriting decisions.
The difference between these technologies becomes clearer when comparing the outputs of underwriting AI with traditional technologies like IDPs. Instead of only extracting every data field, risk assessment solutions that leverage LLMs—like Sixfold— use their trained understanding of what underwriters care about to decide what risk information to summarize and present to underwriters.
This approach differs from IDP by focusing on presenting contextual insights for underwriters, such as risk patterns and appetite alignment. Instead of only providing the extracted data, it highlights key information that directly supports faster decision-making.
Different Approaches to Accuracy
By now, we already know that IDPs and AI solutions aim to improve efficiency and save underwriters time. But what about accuracy? Accuracy is the key component of a successful underwriting decision, which is why evaluating it is so important for tools focused on supporting underwriters. Let’s highlight the differences between what matters for IDP versus AI tools in terms of accuracy.
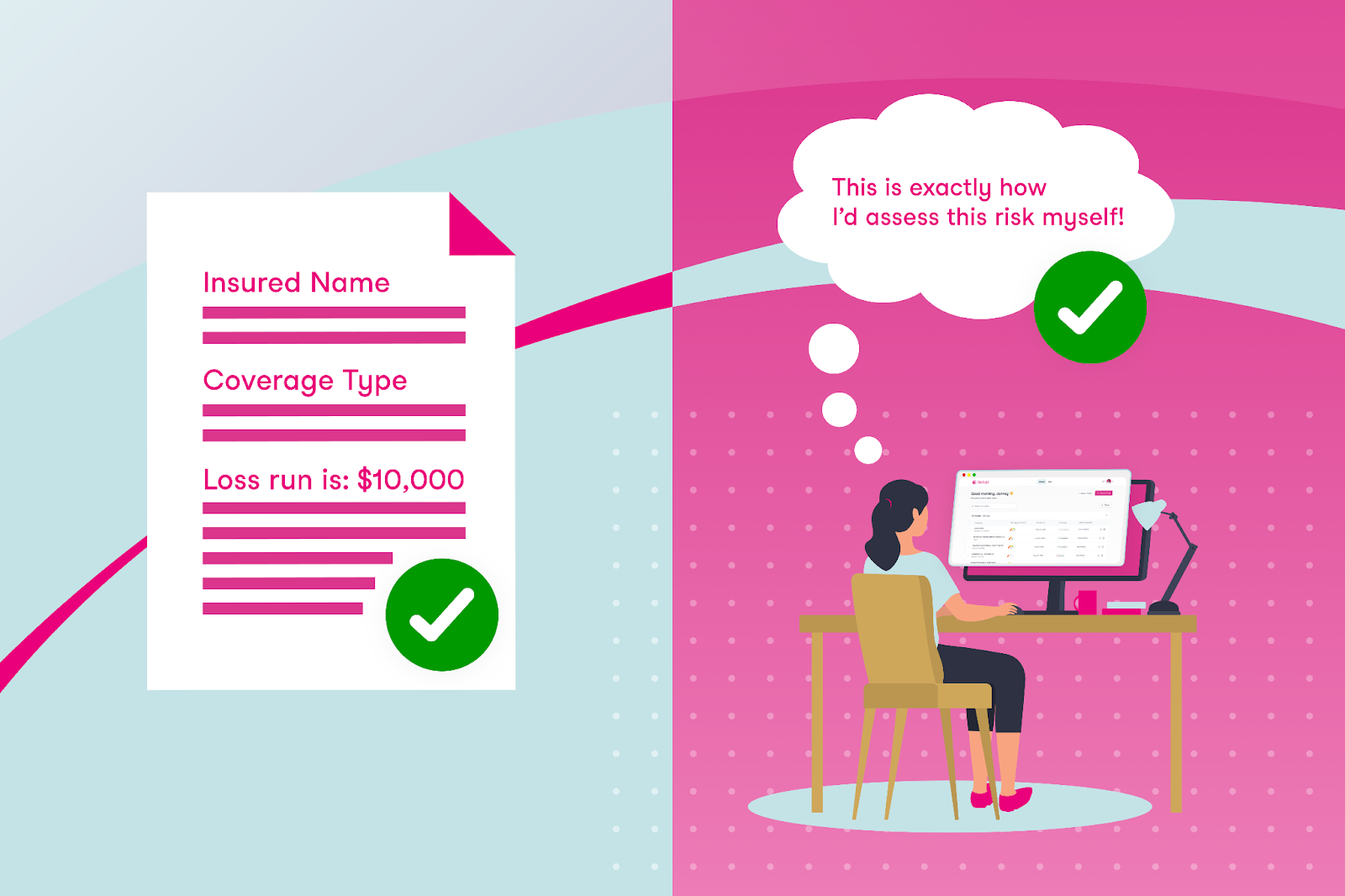
IDPs - Accuracy is about precise field extraction
IDPs focus on data processing, so their success is measured by how accurately they extract each text field. This makes sense given their role in automating structured data collection. If the data field in a document says “Loss run is: $10,000” and the IDP extracts “$1,000” then it’s easy to say that it was not an accurate extraction.
Underwriting AI - Accuracy is about human-like reasoning
The accuracy of underwriting AI lies in their ability to align reasoning and output with the task, presenting the key risk data underwriters themselves would choose to prioritize. Evaluating AI accuracy therefore means determining how closely the AI mirrors an underwriter in assessing risk submissions.
The reality is that, with AI and data extraction, small mistakes don't matter much. For example, consider a loss run: as an underwriter, what’s important isn’t necessarily every individual line or small loss but rather recognizing patterns — such as total losses exceeding $10,000 within a specific timeframe or recurring trends in certain types of losses. An underwriting AI can uncover these insights by focusing on significant trends and aggregating relevant data, looking at a case the same way a human would do.
How to Choose the Right Tool?
The answer isn’t as simple as this or that. There are successful examples of insurers using one, the other, or even both solutions, depending on their needs.
IDP can be a great tool for extracting fields like names, addresses, and other key information from documents to feed into downstream systems. Meanwhile, AI-focused technologies like Sixfold are great for risk analysis. It’s important to note that a company doesn’t need to have an IDP solution in place to adopt an AI solution like Sixfold – these technologies can work independently.
If your goal is to reduce the manual workload for underwriters, start by identifying the exact inefficiencies.
To decide which use of technology is the best fit for your underwriting needs – whether it’s one of these solutions or potentially both – consider the specific challenges you’re addressing. If your goal is to reduce the manual workload for underwriters, start by identifying the exact inefficiencies. For instance, if the issue is that underwriters spend a significant amount of time manually extracting text fields from one document and re-entering it into another system, IDP could be a good option — it’s built for that kind of work, what you are paying for is extracting fields.
However, if you want to address larger inefficiencies in the underwriting process — such as reducing the total time it takes for underwriters to respond to customers — you’ll need to tackle bigger bottlenecks. While it’s true that manual data entry is a challenge, much more of underwriters’ time is spent on manual research tasks such as reviewing hundreds of pages looking for specific risk data, or finding the right NAICS code for a business. These are areas where underwriting AI would be better suited.
So, What's Next for Underwriting?
IDP can efficiently digitize key information, but it doesn’t solve the broader challenges of manual inefficiencies that underwriters deal with daily.
Imagine a future in underwriting where IDP effortlessly extracts core data fields essential for accurate rating, ensuring consistent and reliable input into downstream systems. Meanwhile, specialized underwriting AI takes on the rest of the heavy manual lifting—matching risks to appetite, delivering critical context for decisions, and significantly reducing the time it takes to quote.
By combining the OCR capabilities of IDP with the intelligence of underwriting AI, insurers can make underwriting a lot less manual.
By combining the OCR capabilities of IDP with the intelligence of underwriting AI, insurers can make underwriting a lot less manual. These tools promise a 2025 where underwriters spend less time on repetitive tasks and more time making smart risk decisions.
This post was originally posted on LinkedIn
%20(1500%20x%201000%20px)%20(680%20x%20520%20px).png)
2024 Reel: Sixfold’s Biggest Product Wins And How We Built Them
To close out the year, we sat down with our engineering and product teams for a behind-the-scenes look at what we’ve built. The result? 9 key product achievements.
In case you’re new here, we’ll start by reintroducing ourselves. Sixfold is the first purpose-built AI for insurance underwriting, designed to help insurance underwriters reduce their manual workload. We work with global and innovative insurance carriers like AXIS, Zurich Insurance Group, and Generali.
This year has been full of milestones—we’ve processed over 150,000 submissions, responded to more than 250,000 custom underwriting queries, and gathered 3,200+ hours of underwriter feedback.
So, how did we get here? We sat down with our engineering and product teams to give you an inside look at what we built, how we did it, and the technology behind it all.
The result? Nine of our top product achievements this year, picked by the folks who built them from the ground up, all focused on improving accuracy, efficiency, and transparency for underwriters around the world.
1. Improved Accuracy through Advanced Data Extraction & Risk Matching
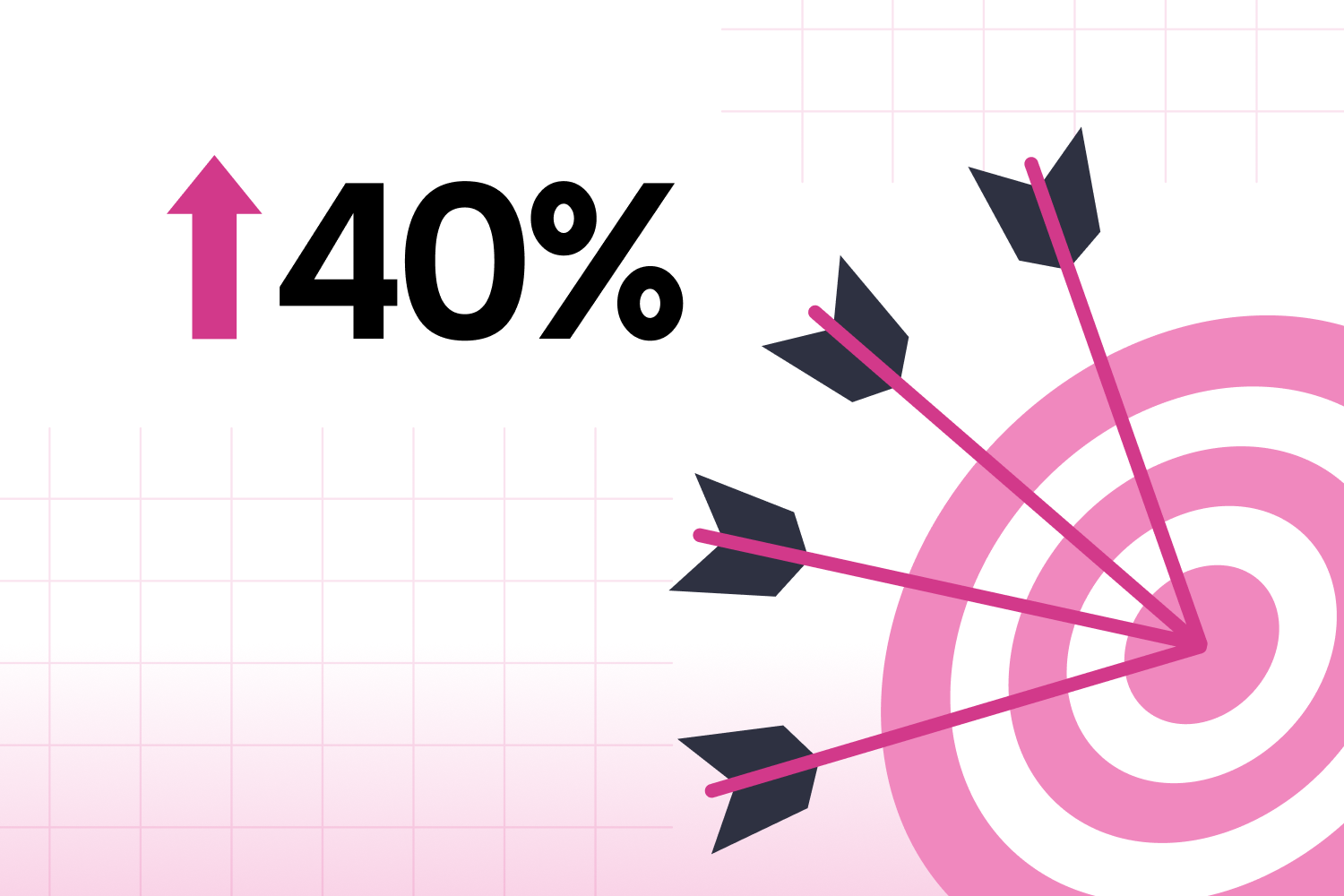
What is it? 💡
Accuracy is one of the key elements at Sixfold and this time we’ve improved our models to deliver:
- Better data extraction from complex insurance documents
- Faster and more accurate matching of insurer risk appetites to key signals within cases
How did we build this? ⚙️
Our AI team focused on these key areas:
- Addressing variability in LLM responses
- Developing accuracy metrics and ensuring targeted output
- Quantitative testing for basic accuracy
“Working with LLMs is unique because they don’t always give the same answer to the same question. To handle that variability, we created metrics to assess how well we extract the necessary information, the precision of our responses, and our performance on key tasks."
- Ian Cook, Head of AI
What’s the impact? 🚀
Boosting accuracy for underwriters is one of our core missions. With our latest models, we’ve seen a 40% improvement in data extraction accuracy compared to six months ago — even from the most difficult-to-read documents.
“General methods to pull text from a PDF don’t go far enough to meet the rigor we demand. By focusing on the specific documents and information underwriters need, we deliver a more targeted, efficient system than off-the-shelf data extraction services.”
- Ian Cook, Head of AI
2. Transparency in One Click Through
In-line Citations
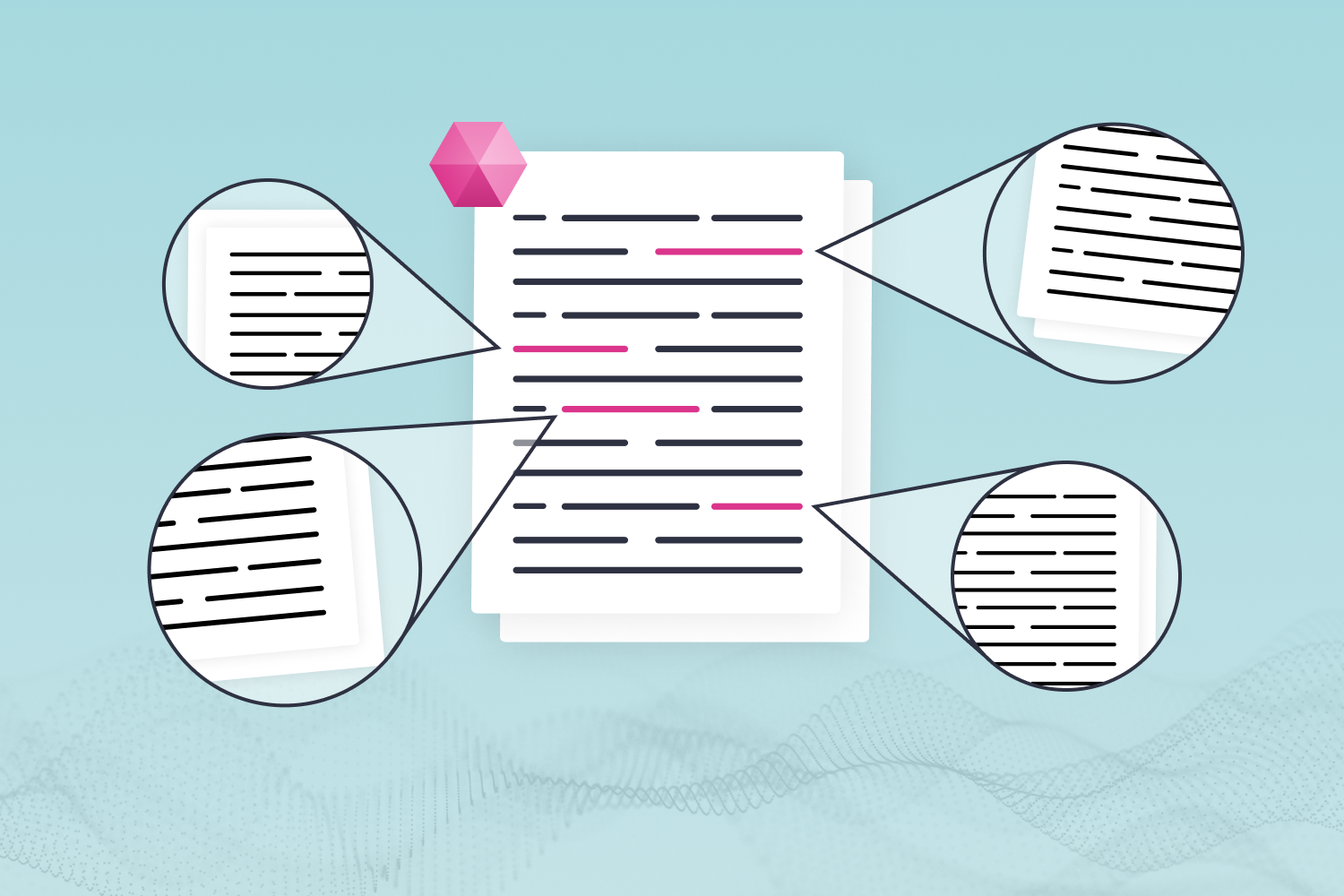
What is it? 💡
With this feature, underwriters can find the source of any information Sixfold is showcasing –with just one click. It’ll show which documents and websites the facts came from. Need to know the exact page? No problem we got you!
In-line citations don’t just build trust in our platform; they also give underwriters context on certain risks and provide full traceability for every piece of risk analysis.
“One of the most important things for an underwriter is not only seeing the pertinent risk signals or questionnaire responses we surface as part of the Sixfold risk analysis but also being able to dig deeper or understand the broader concept behind that information.”
- Lana Jovanovic , Head of Product
How did we build this? ⚙️
In simple terms, we associate each extracted fact with a page number to surface the most relevant information. So, what was the underwriting need behind this development?
- Building trust and confidence in the results
- To quickly locate information without having to read through many documents
“Certain information surfaced as part of that analysis may even trigger additional add-on questions or thoughts that they may have about it, so they can really rapidly be able to dig in further”
- Lana Jovanovic, Head of Product
What’s the impact? 🚀
We’re bringing transparency, speed, and confidence for underwriters—making it that much easier to make informed decisions.
3. Quicker Decisions With Reduced Case Processing Time

What is it? 💡
With our commitment to making underwriters' lives easier, this improvement was an absolute must! Our engineers rolled up their sleeves and worked hard to bring our case processing time down by 61%!
So, what happens during that processing time? Sixfold analyzes aggregated information, searches the web for publicly available information company data, and decides the right NAICS/SIC code classification.
How did we build this? ⚙️
“It started by reviewing our analysis pipeline step-by-step. We measured the processing time and the size of the data payload for each step of our pipeline to see where we could identify any bottlenecks.”
- Ian Hirschfeld, Senior Engineering Manager
Here are the steps we took:
- Broke large data processing tasks into smaller and faster units that could run concurrently
- Reconfigured the pipeline to allow multiple analysis steps to run in parallel
What’s the impact? 🚀
A 61% reduction in processing time—and hopefully, a lot more happy underwriters!
4. Closer Alignment With Your Risk Appetite Through Weighted Risk Signals
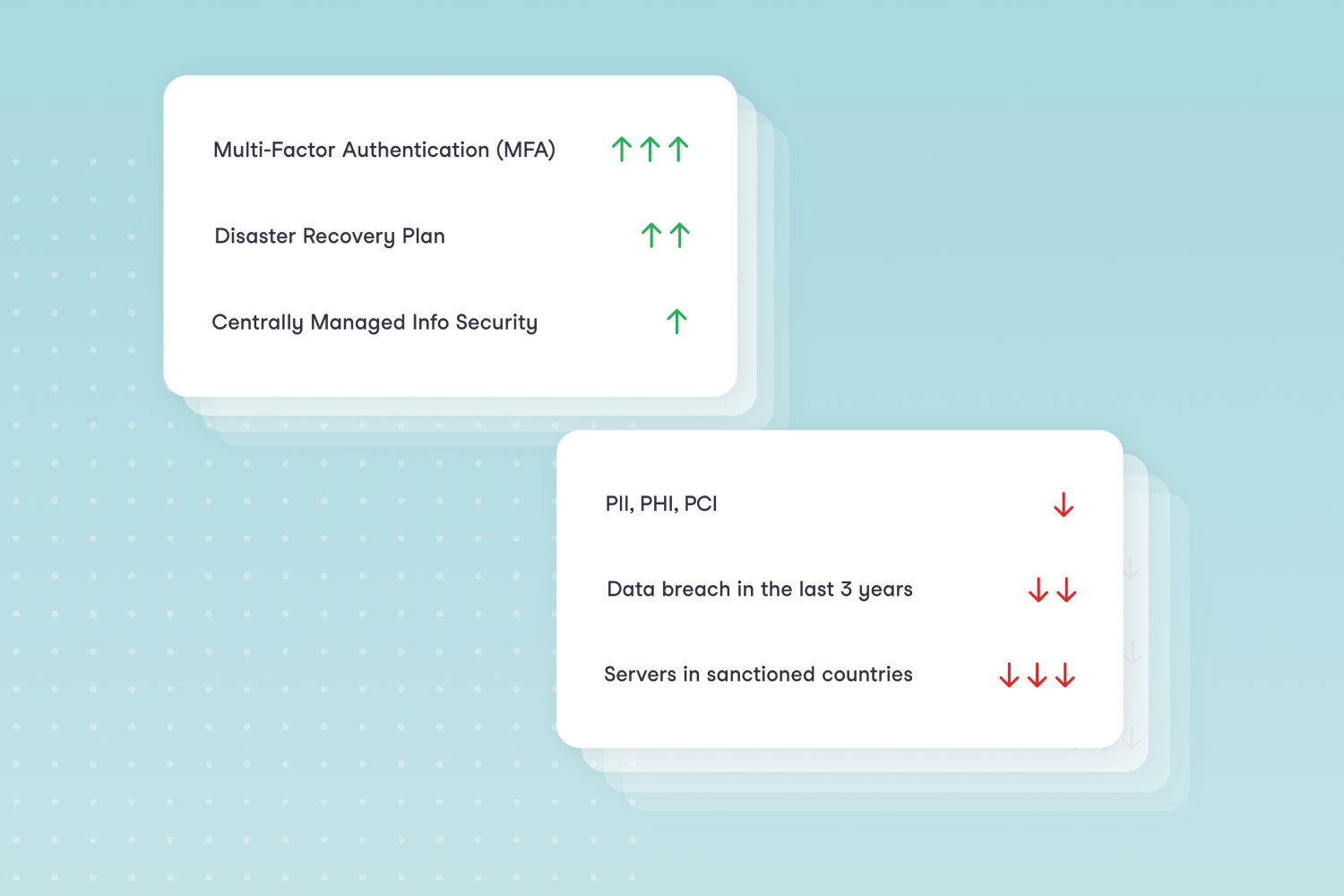
What is it? 💡
This feature will show underwriters all the risk criteria they’ve defined as important and classify those risks as either positive, really positive, negative, or really negative. And yes, the weighted risk signals are tailored to each customer’s unique risk guidelines and definitions.
For example, a cyber company might consider it a “really positive” signal if a prospect has implemented a Multi-Factor Authentication (MFA) process, while not having a backup in place might be something really negative.
How did we build this? ⚙️
Our product team envisioned this feature as something that could easily adapt to each carrier’s unique needs and got close support from our Lloyd's mentors during our 10-week Lloyd’s Lab Program, to refine and improve this feature.
Some of the core elements to figure out when building this feature included:
- Creating a scoring model that’s detailed enough to handle different signals without becoming too complex
- Balancing positive, negative, and neutral signals to accurately reflect real-world underwriting decisions
- Making sure the system could adapt smoothly to each carrier’s existing workflows
Because every carrier and industry interprets risk signals differently, we needed a solution that was both flexible and adaptable.
“The biggest challenge in designing this feature was addressing the diverse UW guidelines across various sectors.”
- Leonardo Momente, Senior Software Engineer
What’s the impact? 🚀
This feature is a great improvement in accuracy in appetite match scoring. This not only saves time but also improves underwriting precision.
“Frequent iteration and testing allowed us to refine the scoring criteria and user interface based on user feedback, ensuring the system was both effective and intuitive.”
- Leonardo Momente, Senior Software Engineer
5. Reliable Evaluations Thanks to Detailed Medical & Lifestyle Insights

What is it? 💡
Life and disability underwriters have to go through hundreds of pages of medical documents, alongside lifestyle details, hobbies, and family medical histories. Sounds like a lot, right? Some might even compare it to detective work.
With our Life and Disability customers in mind, our product and engineering team built the Lifestyle & Medical History Analysis from scratch. This feature analyzes all the documents provided for a case and brings back a summary of all relevant risk information, helping underwriters quote cases more efficiently and quickly.
Sixfold takes care of the heavy lifting so that underwriters can focus on decision-making and relationship-building!
How did we build this? ⚙️
This feature was designed in different phases:
- Sixfold ingests all the documents underwriters upload for a particular case, extracting the relevant text from each file
- We analyze both the structured and unstructured data extracted, summarizing key information for the underwriter
- To make it even easier, we provide a chronological narrative of each case or condition
But, that’s not all! We also flag specific conditions predefined by the carrier and deduplicate repetitive information.
“One challenge was the fact that these medical histories can have a lot of repetitive information. For example, the history of a diagnosis or condition can appear many times across different types of documents.”
- Omeed Fallahi, Software Engineer
What’s the impact? 🚀
This feature dramatically reduces the manual work for underwriters, cutting down processing time for each case.
“You might have a negative risk signal for diabetes, for example. What the AI can do is identify a medication for diabetes that doesn’t explicitly mention the word ‘diabetes’ and flag it as a risk signal. This means the underwriter doesn’t have to constantly check what each medication is and what it treats.”
– Drew Das, Senior AI Engineer
6. Robust Security Through SOC2 Type II Certification + HIPAA Compliance
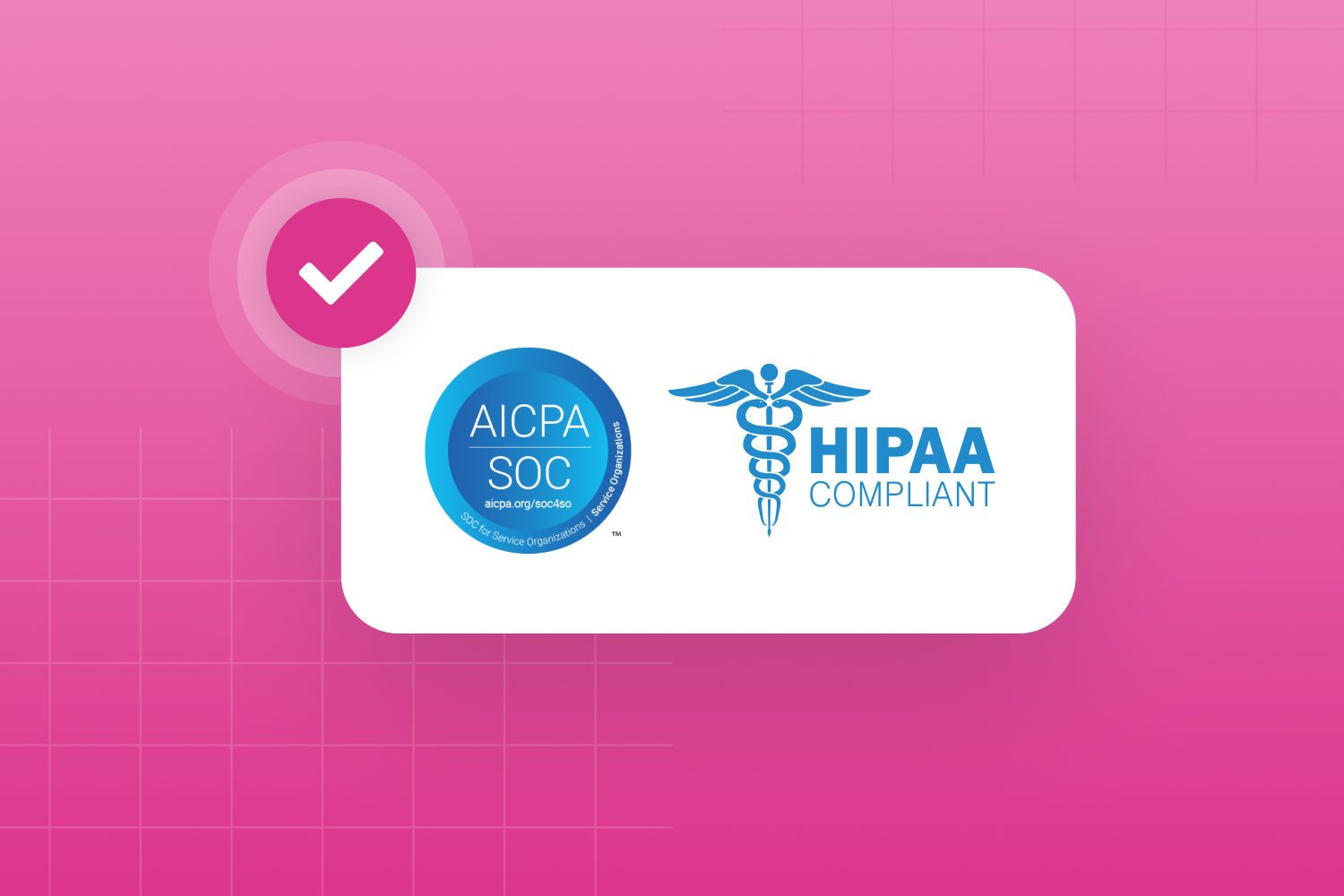
What is it? 💡
Our main goal is to make underwriters' lives easier – that’s at the core of everything we do – making sure our platform plays a huge part in that.
So, what does it mean for Sixfold to be SOC 2 compliant? This means that a neutral, expert third party has evaluated our platform to confirm we meet strict security controls, data protection, and availability standards. With SOC 2, we’ve gone the extra mile to prove these controls work consistently and effectively over time.
And what about HIPAA? HIPAA protects patient health information (PHI), and being HIPAA compliant means we’ve passed an audit confirming that our platform meets the privacy and security requirements needed to serve the life insurance industry.
How did we build this? ⚙️
Over several months, our engineering team addressed a series of steps to align with these regulations:
- Implementing strong access controls, user authentication, and data encryption
- Creating detailed incident response and disaster recovery plans
- Setting up continuous security monitoring, logging, and comprehensive documentation
"Team coordination and implementation proved to be a challenge. We needed to ensure all staff members were properly trained and fully understood our security protocols and compliance requirements. "
- Ryan Garver, Staff Software Engineer
What’s the impact? 🚀
By meeting these standards, we’ve put ourselves in a stronger position to help our customers meet their compliance needs in the insurance industry. It’s all about making Sixfold a trusted, reliable partner for carriers.
“For our platform, SOC 2 Type II and HIPAA compliance are fundamental business necessities, not just regulatory requirements”
- Ryan Garver, Staff Software Engineer
7. Establishing a Responsible AI Framework

What is it? 💡
We love helping underwriters improve their quoting process with AI—that’s our main goal—but we also want to do it in a responsible way and set the benchmark for Responsible AI. With that in mind, we created our Responsible AI Report.
The report gives an overview of Sixfold’s approach to safe AI usage and answers common questions about the topic.
With Sixfold, you can have peace of mind knowing that your data is:
- Never stored or used for training our base model
- Isolated and separated from others
- Locked up tight for ultimate security
How did we build this? ⚙️
Our AI team is constantly working on:
1. Evaluating and re-evaluating our workflows and pipelines
2. Reviewing data that enters our system and assessing it for appropriateness and consistency to ensure it aligns with our Responsible AI principles
3. Refining code, LLM-based interactions, and data governance to ensure no protected class information is inserted or surfaced
“While we always want to make sure we’re aligned with the latest research on Responsible AI, the principles themselves don’t change as often as the tools. Our focus is on applying those principles every time we evaluate new tools and techniques.”
— Ian Cook, Head of AI
What’s the impact? 🚀
The Responsible AI Report reinforces our security standards and compliance commitments, building trust with our customers.
“As someone once said, focus is choosing what not to do. The framework helps us keep focus by making it clear what we should not be doing.”
— Ian Cook, Head of AI
8. Boosting Speed and Accuracy with Fewer Tabs via API
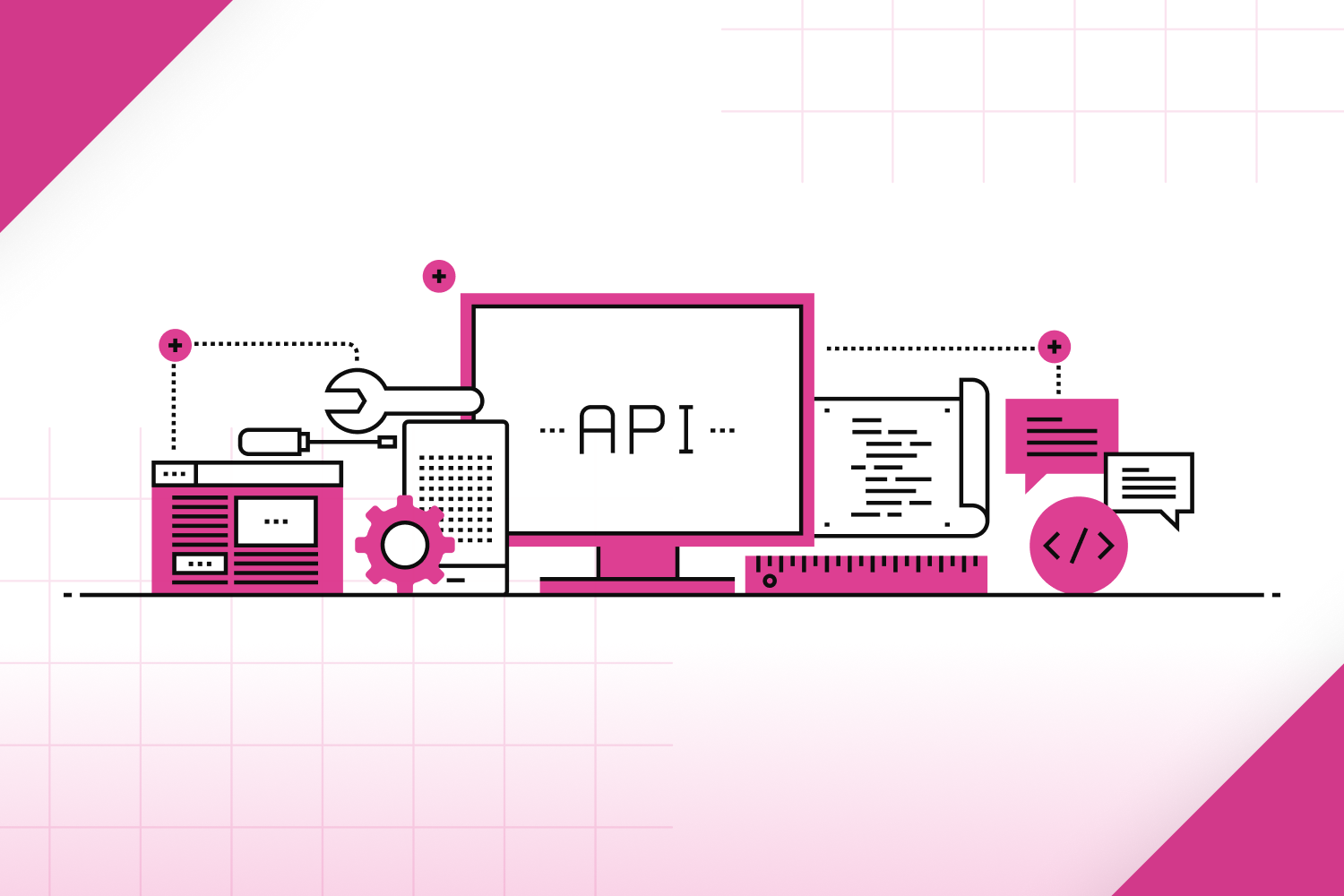
What is it? 💡
You may not even notice, but you’re probably using APIs – Application Programming Interfaces –every day. An API allows different software to communicate while keeping the user on the same interface they’re familiar with. Think about online shopping—you don’t need to leave the site to process your payment through the credit card company, right? That’s an API working behind the scenes!
For Sixfold, launching our API was a big step because it brings simplicity and efficiency to underwriters using our system. Underwriting teams often work across multiple platforms during the quoting process, so we made it easy for our customers’ tech teams to integrate Sixfold’s AI capabilities directly into the tools they’re already using.
How did we build this? ⚙️
Our engineering team focused on:
- Identifying the most critical information to expose through the API and how this information might differ from what is presented in the Sixfold Web UI
- Highlighting the most relevant data, supported by auditable sources
- Minimizing exposure to behind-the-scenes complexity
What’s the impact? 🚀
Sixfold’s API makes it easier for our customers to process a high volume of cases daily.
“One of our customers submits approximately 1,000 cases per day, with an average processing time of 31 seconds per case.”
- Ian Hirschfeld, Senior Engineering Manager
9. Streamlining Workflow Efficiency with SSO Integration

What is it? 💡
Yes, SSO stands for Single Sign-On. But what does that actually mean? We like to think of it as a way to simplify the way users log into applications.
For our customers, that basically means using their corporate email address to securely access Sixfold’s platform. And trust us, implementing SSO isn’t easy—there are plenty of security considerations, and the first thing that comes to mind is compliance!
How did we build this? ⚙️
This feature was a top request from our customers and with a diverse client base, our platform team had a lot of boxes to check:
- Different configurations across different clients
- Which Identity Provider do they use? Microsoft Azure or PingFederate, for example?
- How does user authentication happen—and how do we map users correctly?
“If you have a team of different underwriters, maybe they have different permissions—maybe they can see one case that fits certain criteria but not another.
And when new underwriters join, you want them seamlessly authenticated and authorized the same way as your existing underwriters.”
- Mike Rooney, Principal Software Engineer
What’s the impact? 🚀
No more handling a bunch of passwords! SSO is simply a better way to log in, reducing the chance of leaked credentials.
“Very soon, we’ll just be able to send a link to new customers who want to set up SSO with us. They can go through a detailed onboarding guide at their own pace. Once they’re done and happy with the setup, it turns green on our dashboard and we’re good to go.”
– Mike Rooney, Principal Software Engineer
That’s a 2024 Wrap
Looking back at these product developments and improvements, a few key themes stand out— efficiency, accuracy, and transparency. These are the key elements Sixfold is committed to bringing to underwriters every step of the risk assessment process.
Want to see how we’re making a difference in underwriting today? Check out how AXIS reduced submission time from 30 minutes to just 60 seconds, and how Zurich’s underwriters saved an average of 90 minutes per submission.
Thanks for sticking with us until the end of this post, and we’ll see you in the next one!

Just Launched: Beyond the Policy 🚀
I’m super excited to introduce Beyond the Policy, Sixfold’s innovation hub designed exclusively for underwriters.
Here at Sixfold, we talk to underwriters all the time—on calls, in meetings, at conferences, and through feedback on everything we create. Why? Because understanding the real challenges underwriters face is at the core of what we do.
From those conversations, we realized there’s no central digital space where underwriters can stay updated on industry innovations, exchange insights, and find tools to grow their careers.
That got us thinking — what if we built that place?
So, we did! I’m super excited to introduce Beyond the Policy, Sixfold’s innovation hub designed exclusively for underwriters. Here’s what you can expect:
Real Stories From the Frontlines
Why? Great advice comes from those who’ve faced the same challenges. That’s why Beyond the Policy features interviews with experienced underwriters who share their perspectives on the challenges and opportunities shaping the industry today. These Q&As provide practical insights, lessons learned, and tips you can apply directly to your own work.
We began our interviews with underwriters from major players like Sompo International and NSM, as well as from smaller MGAs and consultancies, ensuring a diverse (and always personal) perspectives.
AI Crash Course for Underwriting Leaders
Why? AI is transforming underwriting, but getting started can feel overwhelming. To help, we’ve created a crash course specifically for underwriting leaders. It’s designed to provide clear, actionable steps for getting started.
This course brings together insights from top AI experts across diverse fields, including the Head of AI at Sixfold and a Lead AI Counsel at Debevoise & Plimpton. Whether you're new to AI or looking to enhance your approach, this resource is a great starting point.
Will we launch more courses in the future? Absolutely - stay tuned for our next one.
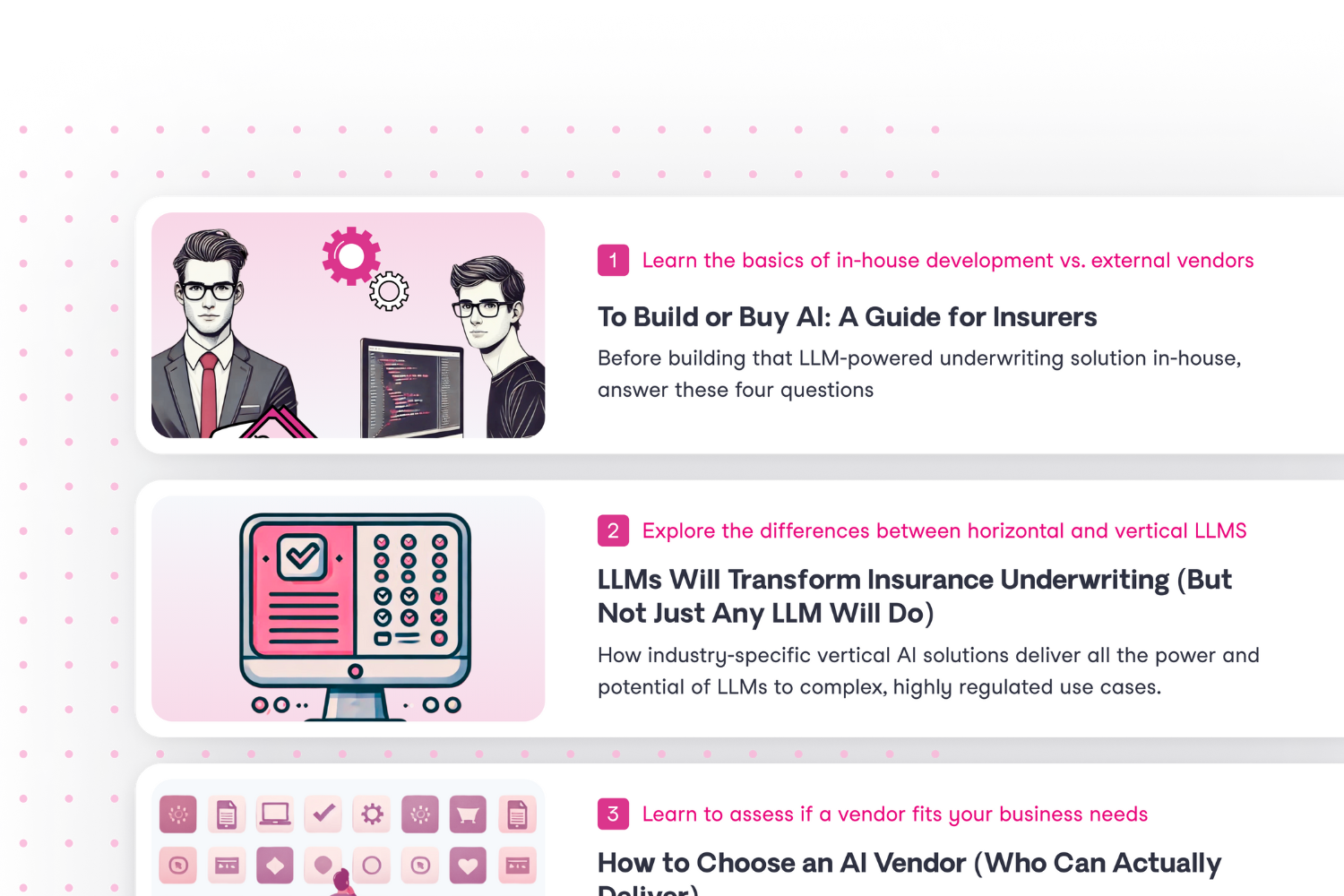
The Best of the Web for Underwriters
Why? The internet is full of information, but finding what’s truly relevant can be a challenge. That’s why we’re doing the work for you. Beyond the Policy features curated content tailored to underwriters, pulling together key industry updates and trends - and updating it frequently. Fresh content, every week!
This week's recommended content includes a great episode from The Insurance Podcast featuring Send (underwriting insurance software) and an article from Deloitte on the insurance outlook in 2025.
Monthly Emails You’ll Actually Want to Read
Why? We know you’re busy, so we’ve packed our monthly emails with the best of what Beyond the Policy has to offer. Expect updates on the latest Q&As, new resources like the AI crash course, and handpicked articles that are worth your time.
These emails are designed to keep you ahead (without adding to your email load).
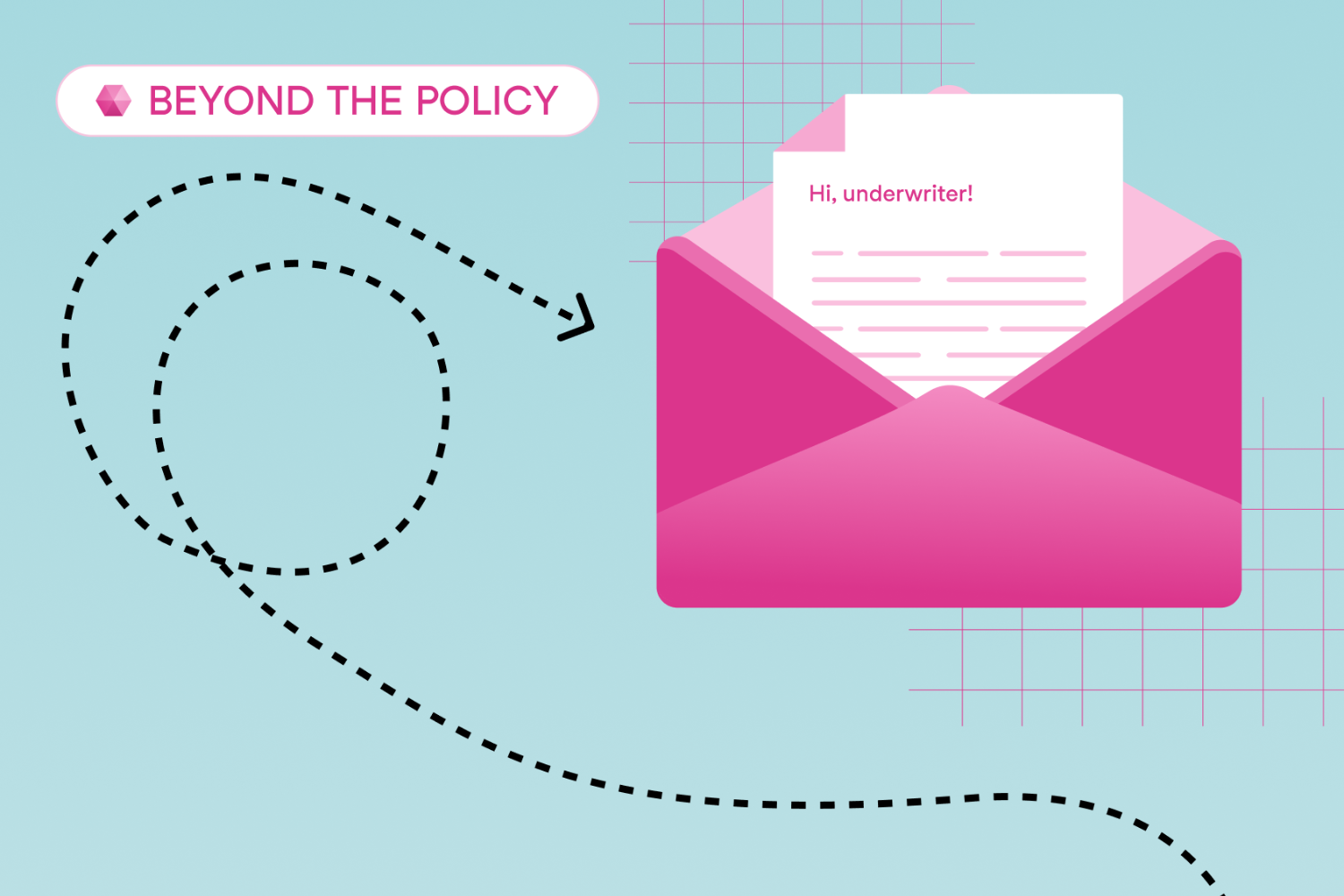
➡️ Beyond the Policy is now live!
If you’re an underwriter, I invite you to check it out, sign up for our monthly emails and hopefully learn a new thing or two.
Enjoy!
.png)
AI Vendor Compliance: A Practical Guide for Insurers
In the hands of insurers, AI can drive great efficiency —safely and responsibly. We recently sat down with Matt Kelly, Data Strategy & Security expert and counsel at Debevoise & Plimpton, to explore how insurers can achieve this.
In the hands of insurers, AI can drive great efficiency —safely and responsibly. We recently sat down with Matt Kelly, Data Strategy & Security expert and counsel at Debevoise & Plimpton, to explore how insurers can achieve this.
Matt has played a key role in developing Sixfold’s 2024 Responsible AI Framework. With deep expertise in AI governance, he has led a growing number of insurers through AI implementations as adoption accelerates across the insurance industry.
To support insurers in navigating the early stages of compliance evaluation, he outlined four key steps:
Step 1: Define the Type of Vendor
Before getting started, it’s important to define what type of AI vendor you’re dealing with. Vendors come in various forms, and each type serves a different purpose. Start by asking these key questions:
- Are they really an AI vendor at all? Practically all vendors use AI (or will do so soon) – even if only in the form of routine office productivity tools and CRM suites. The fact that a vendor uses AI does not mean they use it in a way that merits treating them as an “AI vendor.” If the vendor’s use of AI is not material to either the risk or value proposition of the service or software product being offered (as may be the case, for instance, if a vendor uses it only for internal idea generation, background research, or for logistical purposes), ask yourself whether it makes sense to treat them as an AI vendor at all.
- Is this vendor delivering AI as a standalone product, or is it part of a broader software solution? You need to distinguish between vendors that are providing an AI system that you will interact with directly, versus those who are providing a software solution that leverages AI in a way that is removed from any end users.
- What type of AI technology does this vendor offer? Are they providing or using machine learning models, natural language processing tools, or something else entirely? Have they built or fine-tuned any of their AI systems themselves, or are they simply built atop third-party solutions?
- How does this AI support the insurance carrier’s operations? Is it enhancing underwriting processes, improving customer service, or optimizing operational efficiency?
Pro Tip: Knowing what type of AI solution you need and what the vendor provides will set the stage for deeper evaluations. Map out a flowchart of potential vendors and their associated risks.
Step 2: Identify the Risks Associated with the Vendor
Regulatory and compliance risks are always present when evaluating AI vendors, but it’s important to understand the specific exposures for each type of implementation. Some questions to consider are:
- Are there specific regulations that apply? Based on your expected use of the vendor, are there likely to be specific regulations that would need to be satisfied in connection with the engagement (as would be the case, for instance, with using AI to assist with underwriting decisions in various jurisdictions)?
- What are the data privacy risks? Does the vendor require access to sensitive information – particularly personal information or material nonpublic information – and if so, how do they protect it? Can a customer’s information easily be removed from the underlying AI or models?
- How explainable are their AI models? Are the decision-making processes clear, are they well documented, and can the outputs be explained to and understood by third parties if necessary?
- What cybersecurity protocols are in place? How does the vendor ensure that AI systems (and your data) are secure from misuse or unauthorized access?
- How will things change? What has the vendor committed to do in terms of ongoing monitoring and maintenance? How will you monitor compliance and consistency going forward?
Pro Tip: A good approach is to create a comprehensive checklist of potential risks for evaluation. For each risk that can be addressed through contract terms, build a playbook that includes key diligence questions, preferred contract clauses, and acceptable backup options. This will help ensure all critical areas are covered and allow you to handle each risk with consistency and clarity.
Step 3: Evaluate How Best to Mitigate the Identified Risks
Your company likely has processes in place to handle third-party risks, especially when it comes to data protection, vendor management, and quality control. However, not all risks may be covered, and they may need new or different mitigations. Start by asking:
- What existing processes already address AI vendor risks? For example, if you already have robust data privacy policies, consider whether those policies cover key AI-related risks, and if so, ensure they are incorporated into the AI vendor review process.
- Which risks remain unresolved? Identify the gaps in your current processes to identify unique residual risks – such as algorithmic biases or the need for external audits on AI models – that will require new and ongoing resource allocations.
- How can we mitigate the residual risks? Rather than relying solely on contractual provisions and commercial remedies, consider alternative methods to mitigate residual risks, including data access controls and other technical limitations. For instance, when it comes to sharing personal or other protected data, consider alternative means (including the use of anonymized, pseudonymized, or otherwise abstracted datasets) to help limit the exposure of sensitive information.
Pro Tip: You don’t always need to reinvent the wheel. Look at existing processes within your organization, such as those for data privacy, and determine if they can be adapted to cover AI-specific risks.
Step 4: Establish a Plan for Accepting and Governing Remaining Risks
Eliminating all AI vendor risks cannot be the goal. The goal must be to identify, measure, and mitigate AI vendor risks to a level that is reasonable and that can be accepted by a responsible, accountable person or committee. Keep these final considerations in mind:
- How centralized is your company’s decision-making process? Some carriers may have a centralized procurement team handling all AI vendor decisions, while others may allow business units more autonomy. Understanding this structure will guide how risks are managed.
- Who is accountable for evaluating and approving these risks? Should this decision be made by a procurement team, the business unit, or a senior executive? Larger engagements with greater risks may require involvement from higher levels of the company.
- Which risks are too significant to be accepted? In any vendor engagement, some risks may simply be unacceptable to the carrier. For example, allowing a vendor to resell policyholder information to third parties would often fall into this category. Those overseeing AI vendor risk management usually identify these types of risks instinctively, but clearly documenting them helps ensure alignment among all stakeholders, including regulators and affected parties.
One-Process-Fits-All Doesn’t Apply
As AI adoption grows in insurance, taking a strategic approach can help simplify review processes and prioritize efforts. These four steps provide the foundation for making informed, secure decisions from the start of your AI implementation project.
Evaluating AI vendors is a unique process for each carrier that requires clarity about the type of vendor, understanding the risks, identifying the gaps in your existing processes, and deciding how to mitigate the remaining risks moving forward. Each organization will have a unique approach based on its structure, corporate culture, and risk tolerance.
“Every insurance carrier that I’ve worked with has its own unique set of tools and rules for evaluating AI vendors, what works for one may not be the right fit for another.”
- Matt Kelly, Counsel at Debevoise & Plimpton.