The Role of AI and IDP in Underwriting’s Future

Insurance underwriting has long struggled with a data challenge: finding a way to handle the daily flood of information quickly and accurately. Why? Because the ability to process data significantly impacts the profitability and growth of insurers.
If this sounds familiar, here’s some good news: there’s now a better way to tackle these challenges. Large Language Models (LLMs) are transforming underwriting by processing complex data, providing risk summaries, and delivering tailored risk recommendations—all in ways that were previously unimaginable.
Most of the information underwriters need to make an underwriting decision comes in a mix of structured, semi-structured, and unstructured formats.
Most of the information underwriters need to make an underwriting decision comes in a mix of structured, semi-structured, and unstructured formats, including various types of documents such as broker emails, application forms, and loss runs. This data is often handled manually, consuming 30% to 40% of underwriters' time and ultimately impacting Gross Written Premium (McKinsey & Company).
To address this, insurers have long sought technological solutions. Intelligent Document Processing (IDP) tools were a key step forward, using technologies like Optical Character Recognition (OCR) to extract and organize data. However, while IDP helps digitize information, it doesn’t fully solve the problem of turning data into actionable insights.
Comparing IDP and LLMs
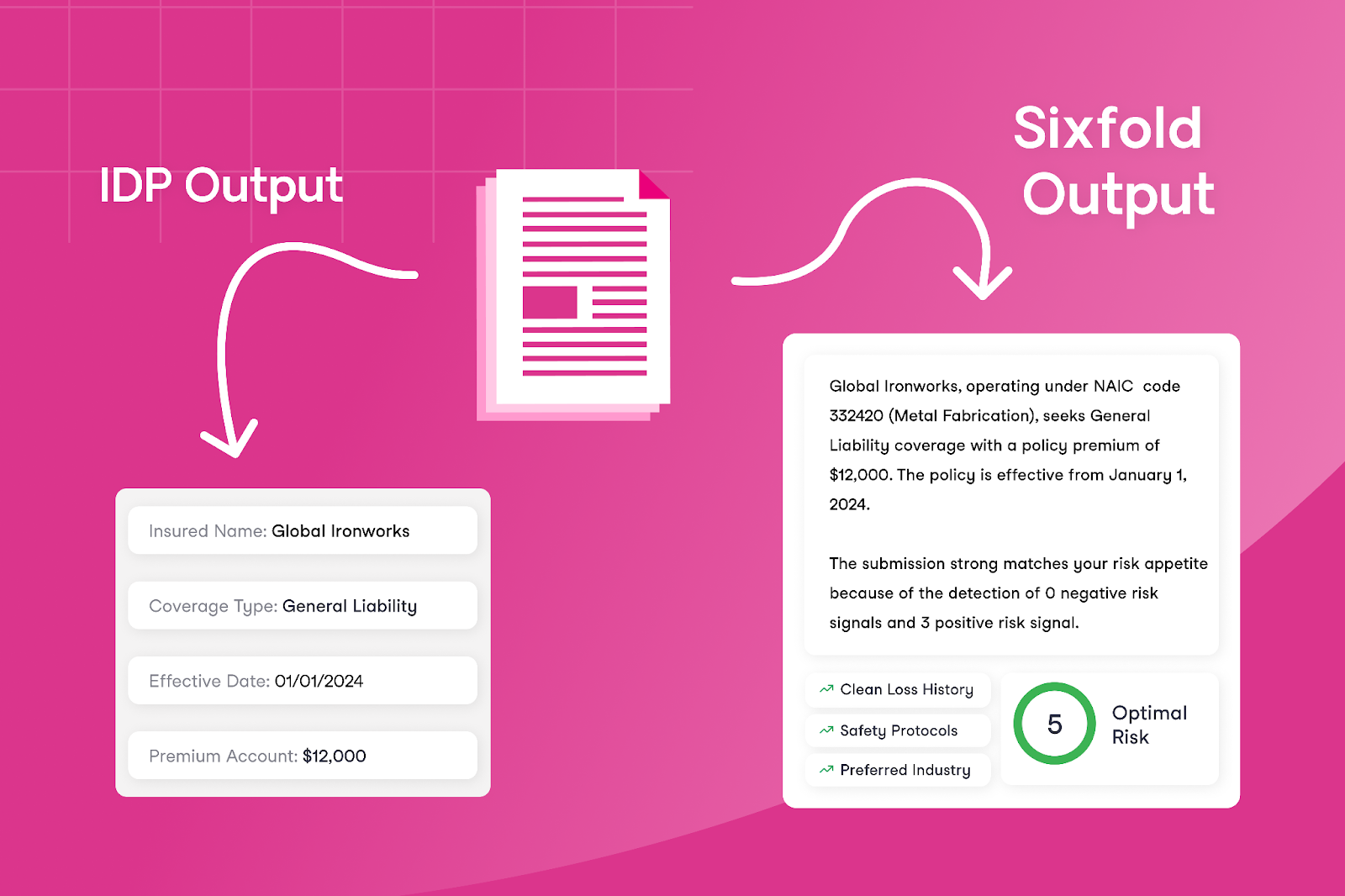
IDPs have been the predominant way of approaching underwriting efficiency, primarily used by insurers to automate tasks in underwriting, policy administration, and claims processing. These tools focus on converting generic documents into structured data by capturing text, classifying document types, and extracting key fields, offering a general solution across different industries.
Digitizing documents - sounds like a no-brainer right? But, what happens if you go beyond simply digitizing documents?
Digitizing documents - sounds like a no-brainer right? But, what happens if you go beyond simply digitizing documents? LLMs make this possible by offering a new approach to improving efficiency and accuracy for underwriters — by not just bringing in all the data but also generating risk analysis and actionable insights. LLMs empower underwriting teams to focus only on the information that truly matters for underwriting decisions.
The difference between these technologies becomes clearer when comparing the outputs of LLMs with traditional technologies like IDPs. Instead of only extracting every data field, risk assessment solutions that leverage LLMs—like Sixfold— use their trained understanding of what underwriters care about to decide what risk information to summarize and present to underwriters.
This approach differs from IDP by focusing on presenting contextual insights for underwriters, such as risk patterns and appetite alignment. Instead of only providing the extracted data, it highlights key information that directly supports faster decision-making.
Different Approaches to Accuracy
By now, we already know that IDPs and LLMs aim to improve efficiency and save underwriters time. But what about accuracy? Accuracy is the key component of a successful underwriting decision, which is why evaluating it is so important for tools focused on supporting underwriters. Let’s highlight the differences between what matters for IDP versus AI tools in terms of accuracy.
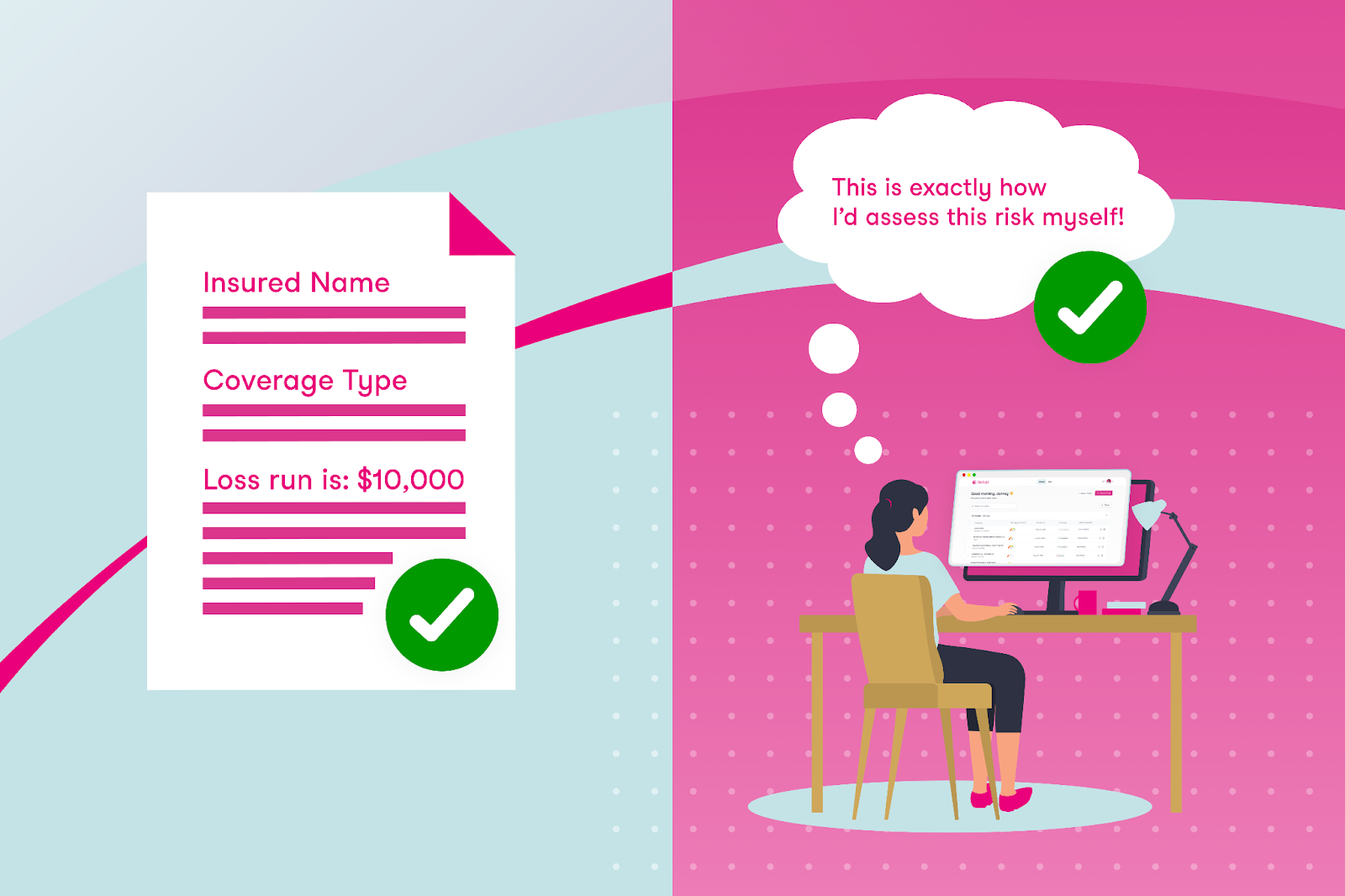
IDPs - Accuracy is about precise field extraction
IDPs focus on data processing, so their success is measured by how accurately they extract each text field. This makes sense given their role in automating structured data collection. If the data field in a document says “Loss run is: $10,000” and the IDP extracts “$1,000” then it’s easy to say that it was not an accurate extraction.
LLMs - Accuracy is about human-like reasoning
The accuracy of LLMs lies in their ability to align reasoning and output with the task, presenting the key risk data underwriters themselves would choose to prioritize. Evaluating LLM accuracy therefore means determining how closely the AI mirrors an underwriter in assessing risk submissions.
The reality is that, with LLMs and data extraction, small mistakes don't matter much. For example, consider a loss run: as an underwriter, what’s important isn’t necessarily every individual line or small loss but rather recognizing patterns — such as total losses exceeding $10,000 within a specific timeframe or recurring trends in certain types of losses. An LLM can uncover these insights by focusing on significant trends and aggregating relevant data, looking at a case the same way a human would do.
How to Choose the Right Tool?
The answer isn’t as simple as this or that. There are successful examples of insurers using one, the other, or even both solutions, depending on their needs.
IDP can be a great tool for extracting fields like names, addresses, and other key information from documents to feed into downstream systems. Meanwhile, LLM technologies like Sixfold are great for risk analysis. It’s important to note that a company doesn’t need to have an IDP solution in place to adopt an AI solution like Sixfold – these technologies can work independently.
If your goal is to reduce the manual workload for underwriters, start by identifying the exact inefficiencies.
To decide which use of technology is the best fit for your underwriting needs – whether it’s one of these solutions or potentially both – consider the specific challenges you’re addressing. If your goal is to reduce the manual workload for underwriters, start by identifying the exact inefficiencies. For instance, if the issue is that underwriters spend a significant amount of time manually extracting text fields from one document and re-entering it into another system, IDP could be a good option — it’s built for that kind of work, what you are paying for is extracting fields.
However, if you want to address larger inefficiencies in the underwriting process — such as reducing the total time it takes for underwriters to respond to customers — you’ll need to tackle bigger bottlenecks. While it’s true that manual data entry is a challenge, much more of underwriters’ time is spent on manual research tasks such as reviewing hundreds of pages looking for specific risk data, or finding the right NAICS code for a business. These are areas where LLMs would be better suited.
So, What's Next for Underwriting?
IDP can efficiently digitize key information, but it doesn’t solve the broader challenges of manual inefficiencies that underwriters deal with daily.
Imagine a future in underwriting where IDP effortlessly extracts core data fields essential for accurate rating, ensuring consistent and reliable input into downstream systems. Meanwhile, specialized LLMs take on the rest of the heavy manual lifting—matching risks to appetite, delivering critical context for decisions, and significantly reducing the time it takes to quote.
By combining the precision of IDP with the intelligence of LLMs, insurers can make underwriting a lot less manual.
By combining the precision of IDP with the intelligence of LLMs, insurers can make underwriting a lot less manual. These tools promise a 2025 where underwriters spend less time on repetitive tasks and more time making smart risk decisions.