Sixfold Resources
Embark on a Journey of Discovery: Uncover a Wealth of Knowledge with Our Diverse Range of Resources.

The Role of AI and IDP in Underwriting’s Future
Underwriting faces a data challenge, with manual processes consuming up to 40% of underwriters’ time. While IDP tools digitize data, underwriting AI goes further—providing actionable insights and enabling smarter risk analysis to improve efficiency and accuracy.
Insurance underwriting has long struggled with a data challenge: finding a way to handle the daily flood of information quickly and accurately. Why? Because the ability to process data significantly impacts the profitability and growth of insurers.
If this sounds familiar, here’s some good news: there’s now a better way to tackle these challenges. Underwriting-focused AI is transforming underwriting by processing complex data, providing risk summaries, and delivering tailored risk recommendations—all in ways that were previously unimaginable.
Most of the information underwriters need to make an underwriting decision comes in a mix of structured, semi-structured, and unstructured formats.
Most of the information underwriters need to make an underwriting decision comes in a mix of structured, semi-structured, and unstructured formats, including various types of documents such as broker emails, application forms, and loss runs. This data is often handled manually, consuming 30% to 40% of underwriters' time and ultimately impacting Gross Written Premium (McKinsey & Company).
To address this, insurers have long sought technological solutions. Intelligent Document Processing (IDP) tools were a key step forward, using technologies like Optical Character Recognition (OCR) to extract and organize data. However, while IDP helps digitize information, it doesn’t fully solve the problem of turning data into actionable insights.
Comparing IDP and Underwriting AI
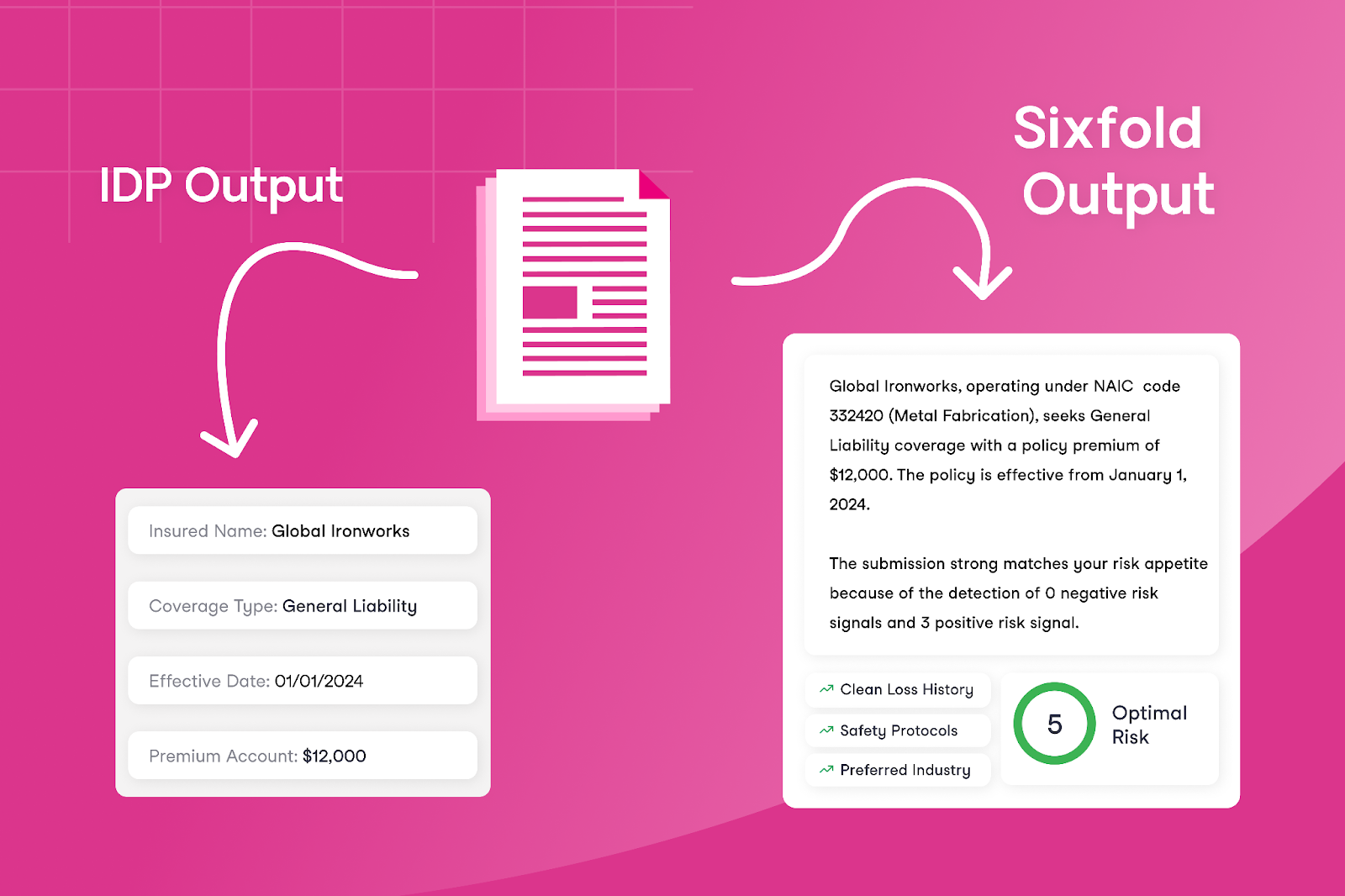
IDPs have been the predominant way of approaching underwriting efficiency, primarily used by insurers to automate tasks in underwriting, policy administration, and claims processing. These tools focus on converting generic documents into structured data by capturing text, classifying document types, and extracting key fields, offering a general solution across different industries.
Digitizing documents - sounds like a no-brainer right? But, what happens if you go beyond simply digitizing documents?
Digitizing documents - sounds like a no-brainer right? But, what happens if you go beyond simply digitizing documents? Underwriting AI makes this possible by offering a new approach to improving efficiency and accuracy for underwriters — by not just bringing in all the data but also generating risk analysis and actionable insights. AI empower underwriting teams to focus only on the information that truly matters for underwriting decisions.
The difference between these technologies becomes clearer when comparing the outputs of underwriting AI with traditional technologies like IDPs. Instead of only extracting every data field, risk assessment solutions that leverage LLMs—like Sixfold— use their trained understanding of what underwriters care about to decide what risk information to summarize and present to underwriters.
This approach differs from IDP by focusing on presenting contextual insights for underwriters, such as risk patterns and appetite alignment. Instead of only providing the extracted data, it highlights key information that directly supports faster decision-making.
Different Approaches to Accuracy
By now, we already know that IDPs and AI solutions aim to improve efficiency and save underwriters time. But what about accuracy? Accuracy is the key component of a successful underwriting decision, which is why evaluating it is so important for tools focused on supporting underwriters. Let’s highlight the differences between what matters for IDP versus AI tools in terms of accuracy.
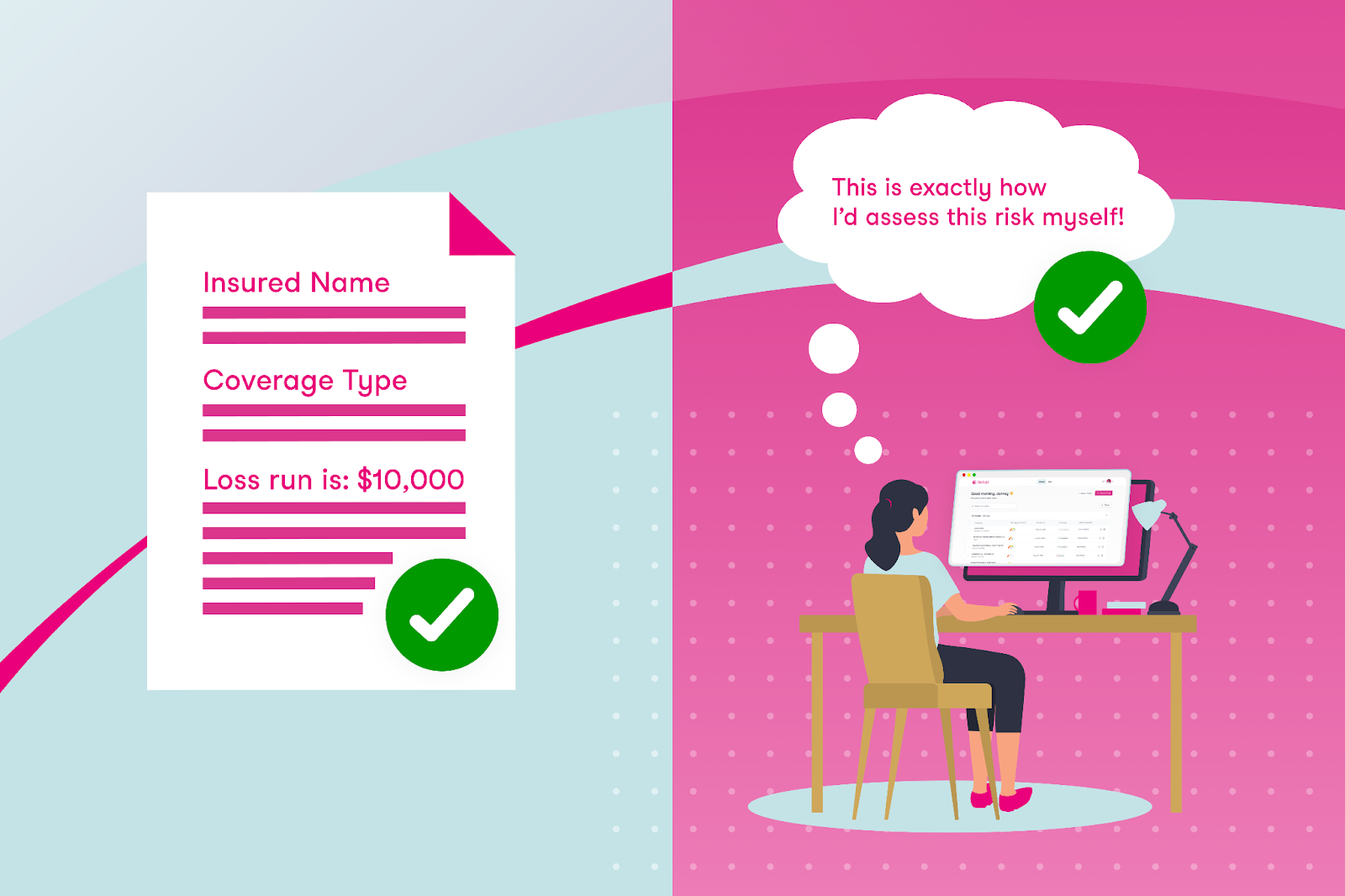
IDPs - Accuracy is about precise field extraction
IDPs focus on data processing, so their success is measured by how accurately they extract each text field. This makes sense given their role in automating structured data collection. If the data field in a document says “Loss run is: $10,000” and the IDP extracts “$1,000” then it’s easy to say that it was not an accurate extraction.
Underwriting AI - Accuracy is about human-like reasoning
The accuracy of underwriting AI lies in their ability to align reasoning and output with the task, presenting the key risk data underwriters themselves would choose to prioritize. Evaluating AI accuracy therefore means determining how closely the AI mirrors an underwriter in assessing risk submissions.
The reality is that, with AI and data extraction, small mistakes don't matter much. For example, consider a loss run: as an underwriter, what’s important isn’t necessarily every individual line or small loss but rather recognizing patterns — such as total losses exceeding $10,000 within a specific timeframe or recurring trends in certain types of losses. An underwriting AI can uncover these insights by focusing on significant trends and aggregating relevant data, looking at a case the same way a human would do.
How to Choose the Right Tool?
The answer isn’t as simple as this or that. There are successful examples of insurers using one, the other, or even both solutions, depending on their needs.
IDP can be a great tool for extracting fields like names, addresses, and other key information from documents to feed into downstream systems. Meanwhile, AI-focused technologies like Sixfold are great for risk analysis. It’s important to note that a company doesn’t need to have an IDP solution in place to adopt an AI solution like Sixfold – these technologies can work independently.
If your goal is to reduce the manual workload for underwriters, start by identifying the exact inefficiencies.
To decide which use of technology is the best fit for your underwriting needs – whether it’s one of these solutions or potentially both – consider the specific challenges you’re addressing. If your goal is to reduce the manual workload for underwriters, start by identifying the exact inefficiencies. For instance, if the issue is that underwriters spend a significant amount of time manually extracting text fields from one document and re-entering it into another system, IDP could be a good option — it’s built for that kind of work, what you are paying for is extracting fields.
However, if you want to address larger inefficiencies in the underwriting process — such as reducing the total time it takes for underwriters to respond to customers — you’ll need to tackle bigger bottlenecks. While it’s true that manual data entry is a challenge, much more of underwriters’ time is spent on manual research tasks such as reviewing hundreds of pages looking for specific risk data, or finding the right NAICS code for a business. These are areas where underwriting AI would be better suited.
So, What's Next for Underwriting?
IDP can efficiently digitize key information, but it doesn’t solve the broader challenges of manual inefficiencies that underwriters deal with daily.
Imagine a future in underwriting where IDP effortlessly extracts core data fields essential for accurate rating, ensuring consistent and reliable input into downstream systems. Meanwhile, specialized underwriting AI takes on the rest of the heavy manual lifting—matching risks to appetite, delivering critical context for decisions, and significantly reducing the time it takes to quote.
By combining the OCR capabilities of IDP with the intelligence of underwriting AI, insurers can make underwriting a lot less manual.
By combining the OCR capabilities of IDP with the intelligence of underwriting AI, insurers can make underwriting a lot less manual. These tools promise a 2025 where underwriters spend less time on repetitive tasks and more time making smart risk decisions.
This post was originally posted on LinkedIn
.png)
AI Vendor Compliance: A Practical Guide for Insurers
In the hands of insurers, AI can drive great efficiency —safely and responsibly. We recently sat down with Matt Kelly, Data Strategy & Security expert and counsel at Debevoise & Plimpton, to explore how insurers can achieve this.
In the hands of insurers, AI can drive great efficiency —safely and responsibly. We recently sat down with Matt Kelly, Data Strategy & Security expert and counsel at Debevoise & Plimpton, to explore how insurers can achieve this.
Matt has played a key role in developing Sixfold’s 2024 Responsible AI Framework. With deep expertise in AI governance, he has led a growing number of insurers through AI implementations as adoption accelerates across the insurance industry.
To support insurers in navigating the early stages of compliance evaluation, he outlined four key steps:
Step 1: Define the Type of Vendor
Before getting started, it’s important to define what type of AI vendor you’re dealing with. Vendors come in various forms, and each type serves a different purpose. Start by asking these key questions:
- Are they really an AI vendor at all? Practically all vendors use AI (or will do so soon) – even if only in the form of routine office productivity tools and CRM suites. The fact that a vendor uses AI does not mean they use it in a way that merits treating them as an “AI vendor.” If the vendor’s use of AI is not material to either the risk or value proposition of the service or software product being offered (as may be the case, for instance, if a vendor uses it only for internal idea generation, background research, or for logistical purposes), ask yourself whether it makes sense to treat them as an AI vendor at all.
- Is this vendor delivering AI as a standalone product, or is it part of a broader software solution? You need to distinguish between vendors that are providing an AI system that you will interact with directly, versus those who are providing a software solution that leverages AI in a way that is removed from any end users.
- What type of AI technology does this vendor offer? Are they providing or using machine learning models, natural language processing tools, or something else entirely? Have they built or fine-tuned any of their AI systems themselves, or are they simply built atop third-party solutions?
- How does this AI support the insurance carrier’s operations? Is it enhancing underwriting processes, improving customer service, or optimizing operational efficiency?
Pro Tip: Knowing what type of AI solution you need and what the vendor provides will set the stage for deeper evaluations. Map out a flowchart of potential vendors and their associated risks.
Step 2: Identify the Risks Associated with the Vendor
Regulatory and compliance risks are always present when evaluating AI vendors, but it’s important to understand the specific exposures for each type of implementation. Some questions to consider are:
- Are there specific regulations that apply? Based on your expected use of the vendor, are there likely to be specific regulations that would need to be satisfied in connection with the engagement (as would be the case, for instance, with using AI to assist with underwriting decisions in various jurisdictions)?
- What are the data privacy risks? Does the vendor require access to sensitive information – particularly personal information or material nonpublic information – and if so, how do they protect it? Can a customer’s information easily be removed from the underlying AI or models?
- How explainable are their AI models? Are the decision-making processes clear, are they well documented, and can the outputs be explained to and understood by third parties if necessary?
- What cybersecurity protocols are in place? How does the vendor ensure that AI systems (and your data) are secure from misuse or unauthorized access?
- How will things change? What has the vendor committed to do in terms of ongoing monitoring and maintenance? How will you monitor compliance and consistency going forward?
Pro Tip: A good approach is to create a comprehensive checklist of potential risks for evaluation. For each risk that can be addressed through contract terms, build a playbook that includes key diligence questions, preferred contract clauses, and acceptable backup options. This will help ensure all critical areas are covered and allow you to handle each risk with consistency and clarity.
Step 3: Evaluate How Best to Mitigate the Identified Risks
Your company likely has processes in place to handle third-party risks, especially when it comes to data protection, vendor management, and quality control. However, not all risks may be covered, and they may need new or different mitigations. Start by asking:
- What existing processes already address AI vendor risks? For example, if you already have robust data privacy policies, consider whether those policies cover key AI-related risks, and if so, ensure they are incorporated into the AI vendor review process.
- Which risks remain unresolved? Identify the gaps in your current processes to identify unique residual risks – such as algorithmic biases or the need for external audits on AI models – that will require new and ongoing resource allocations.
- How can we mitigate the residual risks? Rather than relying solely on contractual provisions and commercial remedies, consider alternative methods to mitigate residual risks, including data access controls and other technical limitations. For instance, when it comes to sharing personal or other protected data, consider alternative means (including the use of anonymized, pseudonymized, or otherwise abstracted datasets) to help limit the exposure of sensitive information.
Pro Tip: You don’t always need to reinvent the wheel. Look at existing processes within your organization, such as those for data privacy, and determine if they can be adapted to cover AI-specific risks.
Step 4: Establish a Plan for Accepting and Governing Remaining Risks
Eliminating all AI vendor risks cannot be the goal. The goal must be to identify, measure, and mitigate AI vendor risks to a level that is reasonable and that can be accepted by a responsible, accountable person or committee. Keep these final considerations in mind:
- How centralized is your company’s decision-making process? Some carriers may have a centralized procurement team handling all AI vendor decisions, while others may allow business units more autonomy. Understanding this structure will guide how risks are managed.
- Who is accountable for evaluating and approving these risks? Should this decision be made by a procurement team, the business unit, or a senior executive? Larger engagements with greater risks may require involvement from higher levels of the company.
- Which risks are too significant to be accepted? In any vendor engagement, some risks may simply be unacceptable to the carrier. For example, allowing a vendor to resell policyholder information to third parties would often fall into this category. Those overseeing AI vendor risk management usually identify these types of risks instinctively, but clearly documenting them helps ensure alignment among all stakeholders, including regulators and affected parties.
One-Process-Fits-All Doesn’t Apply
As AI adoption grows in insurance, taking a strategic approach can help simplify review processes and prioritize efforts. These four steps provide the foundation for making informed, secure decisions from the start of your AI implementation project.
Evaluating AI vendors is a unique process for each carrier that requires clarity about the type of vendor, understanding the risks, identifying the gaps in your existing processes, and deciding how to mitigate the remaining risks moving forward. Each organization will have a unique approach based on its structure, corporate culture, and risk tolerance.
“Every insurance carrier that I’ve worked with has its own unique set of tools and rules for evaluating AI vendors, what works for one may not be the right fit for another.”
- Matt Kelly, Counsel at Debevoise & Plimpton.